How Deep Learning is Used in Military Image Recognition
In recent years, the military has embraced cutting-edge technologies to enhance operational efficiency and security. One of the most transformative advancements has been the integration of deep learning into military image recognition systems. This sophisticated branch of artificial intelligence mimics the way humans learn and interpret information, allowing for the analysis of complex visual data with unprecedented accuracy. Imagine having the ability to sift through thousands of images in mere seconds, pinpointing potential threats or identifying targets with a level of precision that was once thought impossible. Deep learning algorithms are at the forefront of this revolution, enabling military personnel to make informed decisions faster than ever before.
Deep learning is a subset of machine learning that utilizes neural networks to process data. In a military context, it plays a vital role in analyzing vast amounts of image data collected from various sources, such as drones, satellites, and ground surveillance systems. The principles of deep learning are rooted in the ability to recognize patterns and features within images, allowing for the classification and interpretation of complex visual information. By leveraging these technologies, military organizations can enhance their situational awareness, improve reconnaissance efforts, and streamline operational procedures. The relevance of deep learning in defense applications cannot be overstated, as it provides a framework for understanding and responding to dynamic battlefield environments.
Deep learning algorithms have found a plethora of applications within the military sector, significantly enhancing various operations. Some of the most notable use cases include:
- Surveillance: Continuous monitoring of areas of interest to maintain situational awareness.
- Target Identification: Accurate identification of potential threats or enemy combatants.
- Reconnaissance: Gathering intelligence through image analysis to inform strategic decisions.
These applications highlight the versatility of deep learning technologies in military settings. Each use case not only improves operational effectiveness but also aids in making timely and informed decisions, which are critical in high-stakes environments.
Surveillance systems powered by deep learning have revolutionized the way military operations are conducted. These systems enable real-time monitoring of vast areas, providing military personnel with the ability to detect threats before they escalate. Imagine a drone equipped with deep learning capabilities flying over a battlefield, analyzing the terrain and identifying unusual movements. This technology improves situational awareness, allowing for proactive responses and minimizing risks to personnel. The ability to monitor large geographical areas with precision is a game-changer, enhancing operational readiness and effectiveness.
One of the most critical applications of deep learning in military image recognition is automated threat detection. These systems analyze images to identify potential threats, such as enemy vehicles or personnel. The mechanisms behind these systems involve training neural networks on extensive datasets, allowing them to recognize patterns associated with various threats. By automating this process, military forces can significantly reduce the time it takes to assess images and respond to potential dangers, ultimately enhancing the safety of troops and assets.
Another exciting aspect of deep learning in military image recognition is the use of data fusion techniques. These techniques combine information from multiple sources—such as satellite imagery, drone feeds, and ground sensors—to create a more comprehensive understanding of the battlefield. Deep learning facilitates the integration of diverse data types, enabling military analysts to make better-informed decisions. For instance, by fusing data from various sensors, military personnel can achieve a more accurate picture of enemy movements and positions, leading to improved tactical planning.
While the benefits of deep learning in military image recognition are significant, there are also challenges to consider. One major issue is data quality; deep learning algorithms require high-quality, well-labeled datasets for effective training. If the data is poor or biased, the algorithms may produce inaccurate results. Additionally, there is a growing concern about algorithm bias, which can lead to unfair or erroneous outcomes in threat detection. Addressing these challenges is crucial for ensuring the reliability and effectiveness of deep learning technologies in military applications.
The future of deep learning in military image recognition is bright, with emerging trends and advancements on the horizon. As technology continues to evolve, we can expect to see further integration of deep learning with other innovative technologies, such as drones and robotics. This synergy can lead to groundbreaking solutions that enhance military capabilities and operational efficiency.
Integrating deep learning with other technologies like drones and robotics can significantly enhance military capabilities. For example, imagine a drone that not only captures images but also processes them in real-time, identifying threats and relaying information back to command centers. This level of integration can streamline operations, reduce response times, and ultimately save lives. The possibilities are endless as military organizations continue to explore the potential of combining deep learning with other advanced technologies.
As deep learning technologies advance, ethical considerations become paramount. The military's use of artificial intelligence in image recognition raises important moral questions about accountability, privacy, and the potential for misuse. Establishing guidelines for responsible use is essential to ensure that these powerful tools are employed ethically and effectively. As we move forward, it is crucial to balance the benefits of deep learning with the need for ethical oversight and accountability.
- What is deep learning? Deep learning is a subset of artificial intelligence that uses neural networks to analyze and interpret complex data.
- How is deep learning used in military image recognition? It is used for applications such as surveillance, target identification, and reconnaissance, improving operational effectiveness.
- What are the challenges of implementing deep learning in the military? Challenges include data quality, algorithm bias, and the need for robust training datasets.
- What are the future prospects of deep learning in defense? The future looks promising, with potential advancements in integration with drones and robotics, as well as ethical considerations becoming increasingly important.
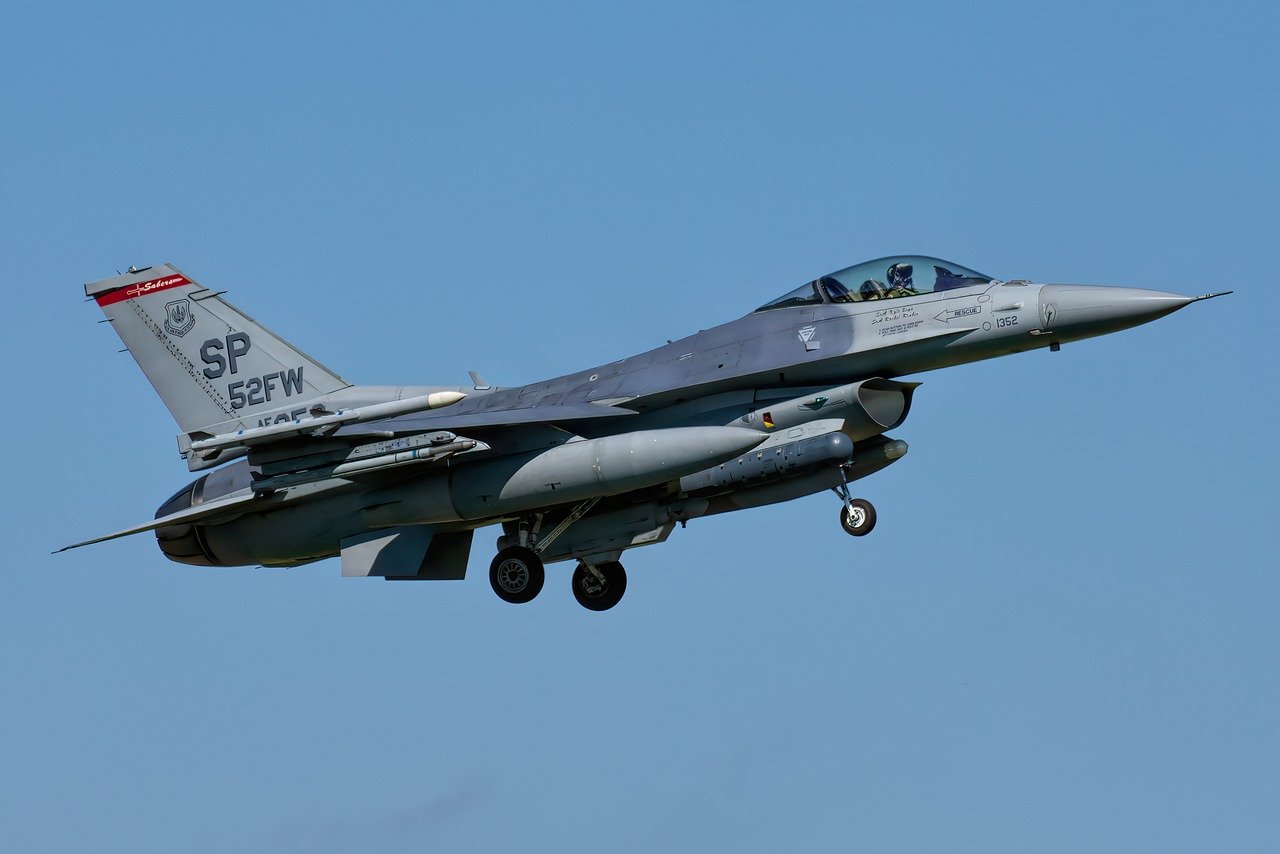
Introduction to Deep Learning in Military
Deep learning has become a game-changer in various fields, and the military is no exception. With its ability to process and interpret complex data sets, deep learning is revolutionizing how military operations are conducted. At its core, deep learning is a subset of artificial intelligence that uses neural networks to mimic the way humans learn from experiences. This technology allows for the analysis of vast amounts of image data, enabling military personnel to make informed decisions rapidly and accurately.
Imagine a world where soldiers can instantly recognize potential threats from aerial imagery or satellite feeds, all thanks to advanced algorithms that can detect patterns and anomalies in real-time. This is not science fiction; it’s happening now. Deep learning models can be trained to identify objects, people, and vehicles in images, which is crucial for reconnaissance missions and operational planning. The military utilizes these capabilities to enhance situational awareness, ensuring that troops are better equipped to respond to threats.
Moreover, the relevance of deep learning extends beyond mere image recognition. It plays a vital role in various military applications, including:
- Surveillance: Continuous monitoring of strategic locations to detect unauthorized activities.
- Target Identification: Accurately identifying enemy targets in complex environments.
- Reconnaissance: Gathering intelligence through automated image analysis.
This technology not only enhances operational efficiency but also significantly reduces the cognitive load on human operators. By automating routine image analysis tasks, deep learning allows military personnel to focus on strategic decision-making rather than getting bogged down in data processing. As we delve deeper into the applications of deep learning in military image recognition, it becomes clear that this technology is not just a tool; it’s a vital asset in modern warfare.
In summary, the integration of deep learning into military operations marks a significant shift in how defense strategies are formulated and executed. As we explore its applications further, we will uncover the profound implications it holds for the future of military intelligence and operational success.
- What is deep learning? Deep learning is a subset of artificial intelligence that uses neural networks to analyze data, mimicking human learning processes.
- How is deep learning used in the military? It is used for image recognition, surveillance, target identification, and reconnaissance, enhancing operational efficiency.
- What are the benefits of using deep learning in military applications? Benefits include improved accuracy in threat detection, reduced cognitive load on personnel, and enhanced decision-making capabilities.
- What challenges does deep learning face in military settings? Challenges include data quality, algorithm bias, and the need for robust training datasets.
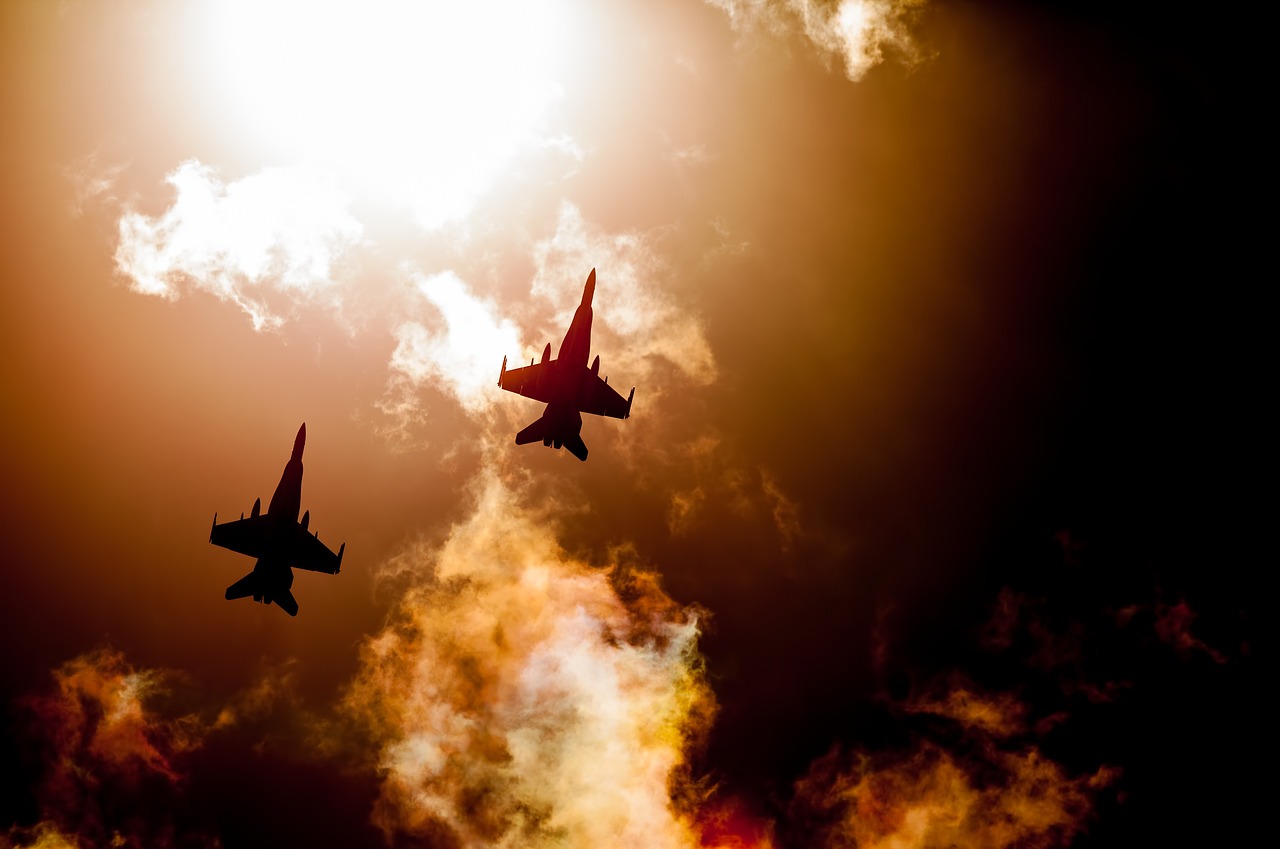
Applications of Deep Learning in Image Recognition
Deep learning has emerged as a game-changer in the realm of military image recognition, significantly enhancing the capabilities of armed forces around the globe. By leveraging advanced algorithms and neural networks, military applications of deep learning have expanded into various critical areas. These include surveillance, target identification, reconnaissance, and even automated threat detection. Each of these applications plays a vital role in ensuring operational effectiveness and decision-making in high-stakes environments.
One of the most prominent applications is in surveillance and monitoring. Imagine a vast battlefield or a border area where every movement matters. Traditional surveillance methods can be slow and prone to human error. However, deep learning algorithms can process vast amounts of visual data in real-time, instantly identifying unusual activities or potential threats. This capability not only enhances situational awareness but also allows military personnel to respond swiftly to emerging situations.
Surveillance systems powered by deep learning enable real-time monitoring of vast areas. These systems utilize convolutional neural networks (CNNs) to analyze imagery captured by drones or stationary cameras. By continuously learning from new data, these algorithms improve their accuracy over time, making them invaluable for military operations. For instance, a drone equipped with deep learning technology can autonomously patrol a designated area, alerting operators to any suspicious activities without human intervention.
In the realm of automated threat detection, deep learning systems are designed to analyze images for potential threats, such as unauthorized vehicles or individuals in restricted zones. These systems work by training on extensive datasets, allowing them to recognize patterns and anomalies effectively. When a potential threat is detected, the system can trigger alerts, enabling military personnel to take immediate action. This capability is crucial for maintaining security in sensitive areas.
Another fascinating aspect of deep learning in military image recognition is the use of data fusion techniques. These methods combine information from multiple sources, such as satellite imagery, drone footage, and ground-based sensors, to enhance image recognition and situational awareness. By integrating diverse data types, military analysts can obtain a more comprehensive understanding of a given scenario. For example, deep learning can correlate data from various sensors to provide a clearer picture of troop movements or enemy positions.
To illustrate the effectiveness of these applications, consider the following table that summarizes the key areas where deep learning is applied in military image recognition:
Application Area | Description | Benefits |
---|---|---|
Surveillance | Real-time monitoring of large areas using drones and cameras | Enhanced situational awareness and swift response |
Automated Threat Detection | Identifying potential threats through image analysis | Increased security and rapid alerts |
Data Fusion | Combining data from various sources for improved insights | Comprehensive understanding of operational environments |
In conclusion, the applications of deep learning in military image recognition are vast and varied, offering significant improvements in operational effectiveness. As technology continues to evolve, we can expect even more innovative uses of deep learning that will shape the future of military strategies.
- What is deep learning? Deep learning is a subset of machine learning that uses neural networks to analyze and interpret complex data.
- How does deep learning improve military image recognition? It enhances the ability to process large volumes of image data quickly and accurately, improving decision-making and operational efficiency.
- What are the challenges of implementing deep learning in military applications? Challenges include data quality, algorithm bias, and the need for robust training datasets.
- What is the future of deep learning in the military? The future looks promising with advancements in technology, integration with drones, and a focus on ethical considerations.
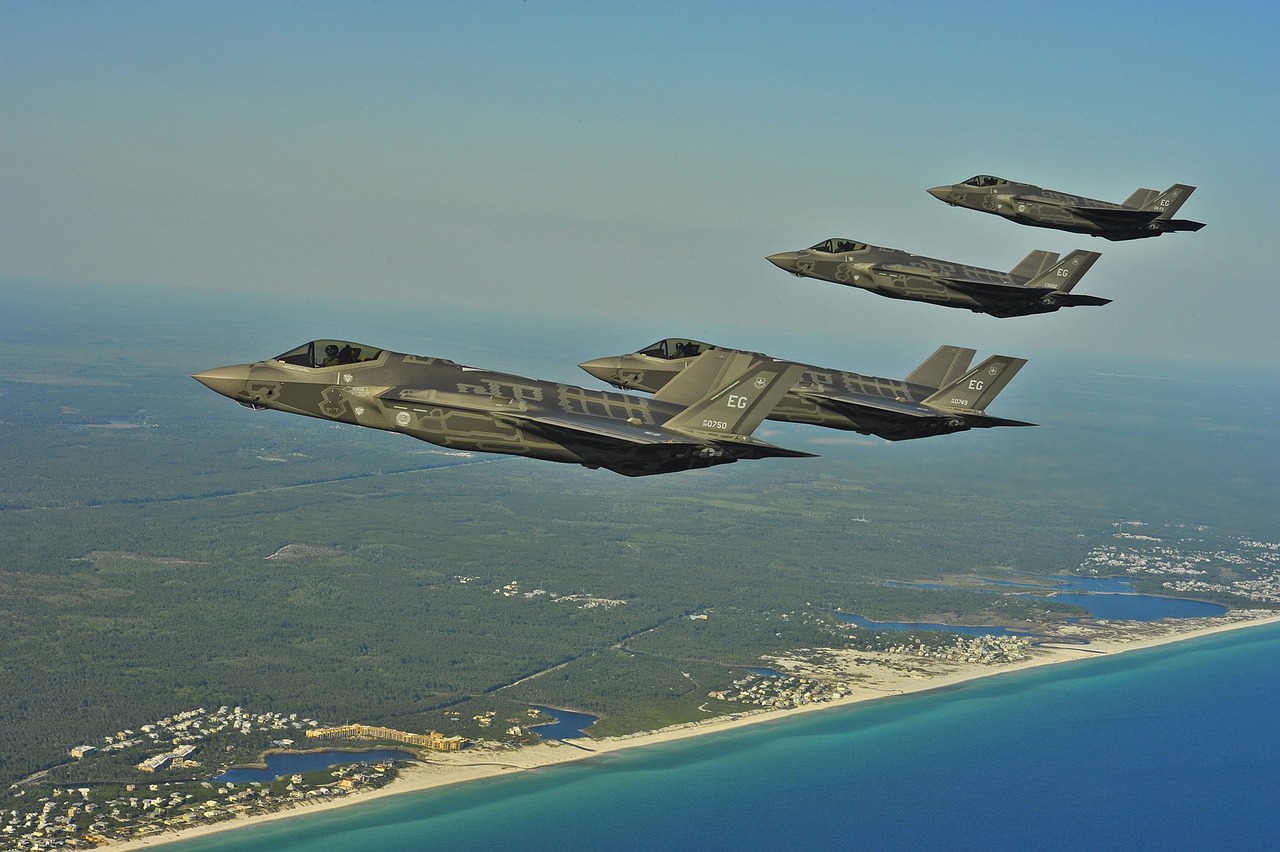
Surveillance and Monitoring
In an era where information is power, have become crucial components of military operations. The infusion of deep learning technologies into these systems has transformed how military forces gather, analyze, and respond to data. Imagine a vast battlefield, where every movement could mean the difference between safety and chaos. Deep learning algorithms act like a vigilant eye, constantly scanning vast areas in real-time, processing images, detecting anomalies, and providing actionable insights.
One of the most remarkable aspects of deep learning in surveillance is its ability to enhance situational awareness. Traditional surveillance methods often relied on human operators to monitor feeds from various sources, which is not only tedious but also prone to errors. With deep learning, machines can analyze thousands of images per second, identifying patterns and unusual activities that might go unnoticed by human eyes. This capability is akin to having a super-soldier who never blinks, tirelessly keeping watch over critical areas.
Furthermore, the use of deep learning in military surveillance systems leads to improved threat detection. For instance, these systems can be trained to recognize specific objects, vehicles, or even individuals, distinguishing between friendly forces and potential threats. This level of precision is vital in high-stakes environments where split-second decisions can save lives. By leveraging vast amounts of data from past encounters, deep learning models can continuously improve their accuracy, making them more effective over time.
To illustrate the effectiveness of deep learning in surveillance, consider the following table that summarizes key features of traditional vs. deep learning-based surveillance systems:
Feature | Traditional Surveillance | Deep Learning Surveillance |
---|---|---|
Data Processing Speed | Slow, relies on human operators | Real-time, automated analysis |
Accuracy | Prone to human error | High, based on trained algorithms |
Scalability | Limited by human resources | Highly scalable with cloud computing |
Adaptability | Static, requires manual updates | Dynamic, continuously learns from new data |
Moreover, deep learning enhances the integration of data from multiple sources, which is critical for effective surveillance. By employing advanced data fusion techniques, military forces can combine information from aerial drones, ground sensors, and satellite imagery to create a comprehensive picture of the operational environment. This holistic view allows commanders to make informed decisions, ensuring that resources are allocated efficiently and effectively.
In conclusion, the role of deep learning in surveillance and monitoring is not just a technological advancement; it’s a revolutionary shift in how military operations are conducted. With the ability to analyze massive amounts of data in real-time, these systems provide the military with a decisive edge on the battlefield. As we continue to explore the potential of deep learning, we can only anticipate even greater advancements that will further enhance our capabilities and ensure the safety of our forces.
- What is deep learning?
Deep learning is a subset of artificial intelligence that uses neural networks to analyze and interpret complex data, including images and videos.
- How does deep learning improve military surveillance?
It enables real-time analysis of vast amounts of data, enhancing threat detection and situational awareness while reducing the likelihood of human error.
- What are data fusion techniques?
These techniques combine information from multiple sources to create a comprehensive view of the operational environment, aiding decision-making processes.
- What challenges exist in implementing deep learning in military applications?
Challenges include data quality, algorithm bias, and the need for robust training datasets to ensure accuracy and reliability.
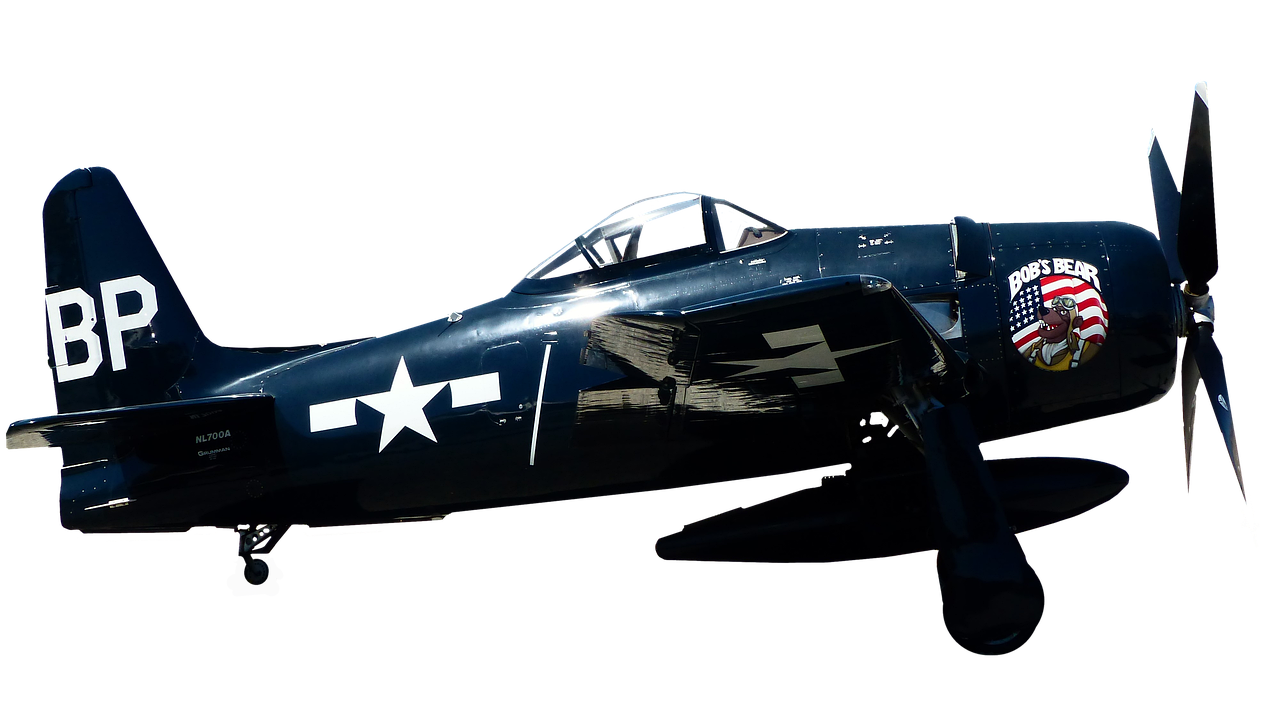
Automated Threat Detection
In the rapidly evolving landscape of military operations, powered by deep learning is becoming a game-changer. Imagine a system that can analyze thousands of images in a matter of seconds, identifying potential threats with remarkable precision. This is not just a futuristic concept; it's a reality thanks to the advancements in artificial intelligence and machine learning. Automated threat detection systems utilize sophisticated algorithms that are trained on vast datasets, allowing them to recognize patterns and anomalies that may indicate a security risk.
At the heart of these systems lies a deep learning architecture known as a convolutional neural network (CNN). CNNs are designed to process pixel data and can effectively learn from the visual features present in images. For instance, in a military context, these networks can be trained to identify various objects, such as vehicles, weapons, or even unusual movements in a surveillance video. The ability to differentiate between a friend and a foe in real-time is crucial for maintaining operational security and ensuring the safety of personnel.
One of the standout features of automated threat detection is its real-time processing capability. Traditional methods of threat analysis often involve human operators sifting through hours of footage, which can lead to delays and missed opportunities. However, with deep learning, systems can continuously monitor feeds from drones, satellites, or ground-based cameras, providing commanders with immediate insights. This not only enhances situational awareness but also enables quicker decision-making on the battlefield.
Moreover, the integration of data fusion techniques amplifies the effectiveness of automated threat detection. By combining data from various sources—like radar, thermal imaging, and optical cameras—these systems create a comprehensive picture of the operational environment. This fusion of information allows for more accurate threat assessments and reduces the likelihood of false positives, which can be detrimental in high-stakes scenarios.
However, while the benefits are substantial, there are also challenges associated with automated threat detection. Issues such as algorithm bias can arise if the training datasets are not diverse enough, potentially leading to misidentification of threats. Addressing these challenges requires ongoing research and development to ensure that these systems are both reliable and fair. As military organizations continue to adopt these technologies, it is imperative that they also focus on establishing robust guidelines for their implementation.
In conclusion, automated threat detection represents a significant leap forward in military image recognition. By harnessing the power of deep learning, these systems not only enhance operational efficiency but also improve the safety of military personnel. As technology continues to advance, we can expect even more innovative solutions that will redefine how threats are identified and addressed in real-time.
- What is automated threat detection?
Automated threat detection refers to the use of advanced algorithms and deep learning technologies to identify potential threats in real-time, enhancing situational awareness in military operations. - How does deep learning improve threat detection?
Deep learning algorithms, particularly convolutional neural networks (CNNs), can analyze vast amounts of image data quickly and accurately, allowing for better identification of objects and anomalies. - What are the challenges of automated threat detection?
Challenges include algorithm bias, the need for diverse training datasets, and ensuring the reliability of the systems in high-pressure situations. - How does data fusion enhance threat detection?
Data fusion combines information from multiple sources, such as radar and thermal imaging, to create a more comprehensive view of the environment, improving accuracy in threat assessment.
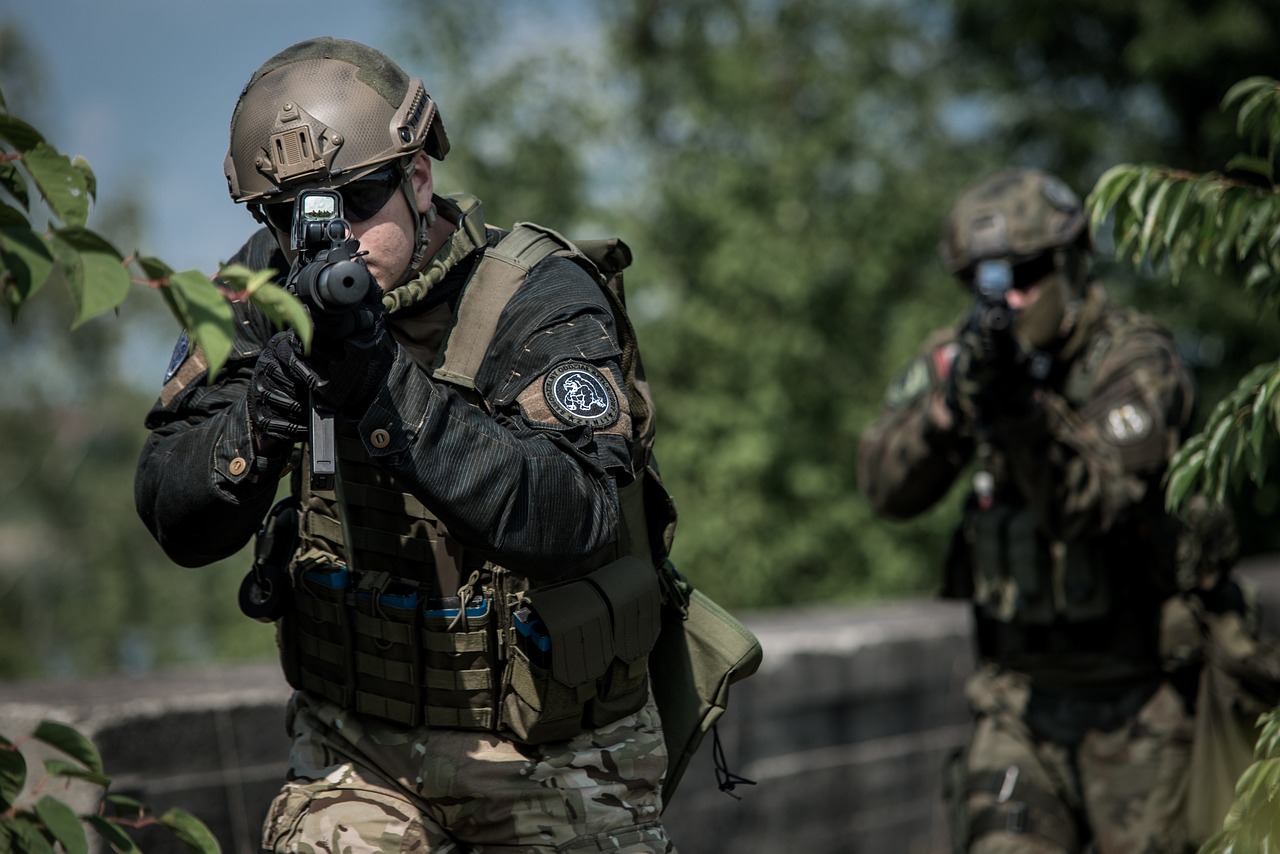
Data Fusion Techniques
In the realm of military image recognition, play a pivotal role in enhancing the accuracy and reliability of intelligence gathered from various sources. Imagine trying to solve a complex puzzle where each piece represents a different type of data—satellite imagery, drone footage, ground sensor information, and even human intelligence reports. By integrating these diverse data types, military analysts can create a more comprehensive picture of the battlefield, leading to better-informed decisions.
Data fusion combines information from multiple sources to produce a more accurate and complete representation of the operational environment. This process is crucial in military applications where real-time decision-making can mean the difference between success and failure. The effectiveness of data fusion relies heavily on deep learning algorithms, which can analyze vast amounts of data quickly and efficiently. These algorithms can identify patterns and correlations that might go unnoticed by human analysts.
One of the most significant advantages of using deep learning for data fusion is its ability to handle unstructured data. Traditional methods often struggle with the sheer volume and variety of data generated in military operations. However, deep learning models can process unstructured data—such as images and video—alongside structured data, like numerical sensor readings. This capability allows for a more nuanced analysis of the operational landscape.
To illustrate the process, consider the following table that summarizes the types of data typically fused in military applications:
Data Type | Description | Example Usage |
---|---|---|
Satellite Imagery | High-resolution images captured from space | Mapping enemy territory |
Drone Footage | Real-time video feeds from unmanned aerial vehicles | Surveillance of troop movements |
Ground Sensors | Data collected from various sensors deployed on the ground | Detecting movement or changes in the environment |
Human Intelligence | Reports and observations from personnel on the ground | Gathering insights on enemy behavior |
Moreover, deep learning facilitates the real-time analysis of this fused data, allowing military personnel to respond swiftly to emerging threats. For instance, if a drone detects unusual activity in a specific area, the deep learning algorithms can quickly analyze satellite images and ground sensor data to confirm the nature of the activity. This rapid processing can lead to timely interventions, ultimately enhancing mission success rates.
However, it’s essential to note that while data fusion techniques offer significant advantages, they also come with challenges. Issues such as data quality, algorithm bias, and the integration of legacy systems can complicate the fusion process. Therefore, continuous improvement of algorithms and robust training datasets are crucial to maximizing the benefits of data fusion in military operations.
In conclusion, data fusion techniques, powered by deep learning, are transforming military image recognition. By enabling the integration of diverse data sources and providing real-time insights, these technologies not only enhance operational effectiveness but also pave the way for innovative military strategies.
- What is data fusion in military applications? Data fusion refers to the integration of data from multiple sources to create a comprehensive understanding of a situation, enhancing decision-making in military operations.
- How does deep learning improve data fusion? Deep learning algorithms can analyze vast amounts of unstructured and structured data quickly, identifying patterns and correlations that improve the accuracy of military intelligence.
- What types of data are typically fused in military operations? Common data types include satellite imagery, drone footage, ground sensor data, and human intelligence reports.
- What challenges are associated with data fusion? Challenges include ensuring data quality, addressing algorithm bias, and integrating new technologies with existing systems.

Challenges in Implementing Deep Learning
While the potential of deep learning in military image recognition is vast, there are significant challenges that must be addressed to fully realize its capabilities. One of the primary issues is data quality. Deep learning algorithms rely heavily on large datasets to learn and make accurate predictions. However, in military contexts, obtaining high-quality, labeled data can be a daunting task. The data may come from various sources, each with its own level of reliability, leading to inconsistencies that can hinder the effectiveness of the algorithms.
Another major concern is algorithm bias. If the training data contains biases—whether intentional or unintentional—the algorithms will learn and perpetuate these biases in their predictions. This can have serious implications in military operations, where the stakes are incredibly high. For instance, biased algorithms may misidentify threats or fail to recognize friendly units, potentially leading to catastrophic outcomes.
Moreover, the need for robust training datasets cannot be overstated. Creating and maintaining these datasets requires significant resources, both in terms of time and finances. Military organizations must invest in data collection, processing, and continuous updates to ensure that their deep learning systems are learning from the most current and relevant information available.
In addition to these technical challenges, there are also operational hurdles to consider. Integrating deep learning systems into existing military frameworks demands a shift in how personnel are trained and how operations are conducted. Military staff must be equipped with the necessary skills to work alongside these advanced technologies, which may require extensive retraining and adaptation.
Lastly, cybersecurity poses a significant risk. As deep learning systems become more integrated into military operations, they also become more vulnerable to cyberattacks. Ensuring the integrity and security of these systems is critical, as any breach could lead to compromised data and operational failures.
In summary, while deep learning holds great promise for enhancing military image recognition, addressing these challenges is essential for successful implementation. Overcoming issues related to data quality, algorithm bias, training datasets, operational integration, and cybersecurity will be key to harnessing the full potential of this transformative technology.
- What is deep learning? Deep learning is a subset of machine learning that uses neural networks with multiple layers to analyze and interpret complex data.
- How does deep learning improve military image recognition? Deep learning enhances military image recognition by enabling automated analysis of vast amounts of image data, leading to quicker and more accurate threat detection.
- What are the risks associated with using deep learning in the military? Risks include data quality issues, algorithm bias, cybersecurity threats, and the need for significant training and adaptation for military personnel.
- How can biases in algorithms be mitigated? Regular audits of training datasets, diverse data sourcing, and ongoing monitoring of algorithm performance can help mitigate biases.

Future Prospects of Deep Learning in Defense
As we look ahead, the future of deep learning in military image recognition is not just promising; it's teeming with potential that could redefine the landscape of defense strategies. Imagine a world where machines can not only recognize threats but also predict them before they materialize. This is not science fiction; it's the impending reality shaped by advancements in artificial intelligence (AI) and deep learning technologies. The integration of these technologies into military operations can lead to enhanced decision-making processes, improved situational awareness, and ultimately, a more secure environment for both military personnel and civilians.
One of the most exciting prospects lies in the integration of deep learning with other technologies. For instance, when paired with drones and robotics, deep learning can significantly enhance reconnaissance missions. Drones equipped with advanced image recognition capabilities can autonomously monitor vast areas, identifying potential threats while minimizing human error. This synergy between deep learning and unmanned systems can lead to faster response times and more accurate threat assessments.
Moreover, the development of adaptive algorithms that learn from their environment can revolutionize how military operations are conducted. These algorithms can analyze real-time data from various sensors, allowing for a more comprehensive understanding of the battlefield. For example, a drone might not only identify enemy positions but also adapt its surveillance pattern based on the movements of those targets, thereby improving the effectiveness of military strategies.
However, the future isn't without its challenges. As deep learning technologies evolve, the need for robust training datasets becomes increasingly critical. Military operations often operate in dynamic and unpredictable environments, which means that training data must be constantly updated to reflect real-world conditions. This requirement raises questions about data collection, privacy, and the ethical implications of using AI in defense scenarios.
Speaking of ethics, as deep learning continues to advance, ethical considerations will play a crucial role in shaping its application in military contexts. The potential for misuse or unintended consequences of AI-driven technologies necessitates a framework of guidelines for responsible use. Establishing these guidelines will ensure that while we harness the power of deep learning, we also uphold the values of accountability and integrity in military operations.
In conclusion, the future of deep learning in defense is not just about technology; it's about the profound impact it can have on military strategies and operational efficiency. As we continue to explore these advancements, we must also remain vigilant about the ethical implications, ensuring that the power of AI is used responsibly. With the right balance, deep learning could very well be the key to a safer, more secure world.
- What is deep learning in military applications?
Deep learning refers to a subset of artificial intelligence that uses neural networks to analyze and interpret complex data, such as images, in military contexts for enhanced decision-making and operational effectiveness.
- How can deep learning improve military surveillance?
Deep learning algorithms can process vast amounts of visual data in real-time, enabling automated threat detection and improving situational awareness during military operations.
- What are the ethical concerns surrounding AI in the military?
Ethical concerns include the potential for misuse of AI technologies, the need for accountability in decision-making, and the implications of using autonomous systems in combat scenarios.

Integration with Other Technologies
In the rapidly evolving landscape of military operations, the integration of deep learning with other advanced technologies is not just a trend; it’s a necessity. Imagine a battlefield where drones equipped with sophisticated image recognition capabilities can analyze vast terrains in real-time, identifying potential threats and providing actionable intelligence to ground troops. This is not science fiction; it’s the future of military strategy, driven by the synergy between deep learning and other technologies.
One of the most significant integrations is with unmanned aerial vehicles (UAVs). These drones, when powered by deep learning algorithms, can autonomously navigate complex environments while simultaneously processing imagery to detect and classify objects. The result? Enhanced situational awareness that allows military personnel to make informed decisions swiftly. For instance, a drone can distinguish between civilian vehicles and potential threats, significantly reducing the risk of collateral damage.
Moreover, the combination of deep learning with robotics is paving the way for innovative solutions in military logistics and reconnaissance. Robots equipped with deep learning capabilities can autonomously scout areas, gather intelligence, and even perform tasks that are too dangerous for human soldiers. This not only enhances operational efficiency but also preserves human life in high-risk scenarios.
Another exciting avenue is the integration of deep learning with Internet of Things (IoT) devices. In a military context, IoT sensors can collect data from various sources, such as weather conditions, troop movements, and equipment statuses. When this data is analyzed using deep learning algorithms, military strategists can gain comprehensive insights into the battlefield environment. For example, a network of IoT sensors could alert commanders to changes in enemy movements or environmental conditions, enabling quicker responses and strategic adjustments.
However, while the integration of these technologies offers immense potential, it also presents challenges. The complexity of merging different systems can lead to compatibility issues, and the reliance on automated systems raises questions about accountability and control. As military operations increasingly depend on these integrated technologies, addressing these challenges will be crucial to ensuring their effectiveness and reliability.
In conclusion, the integration of deep learning with other technologies like drones, robotics, and IoT is revolutionizing military image recognition and operational strategies. As these technologies continue to evolve, we can expect even more innovative solutions that enhance the capabilities of military forces around the globe.
- What is deep learning?
Deep learning is a subset of machine learning that uses neural networks to analyze and interpret complex data. In the military, it is used for tasks like image recognition and threat detection. - How do drones enhance military operations?
Drones equipped with deep learning can autonomously analyze images and data, improving situational awareness and decision-making in real-time. - What are the ethical considerations of using AI in the military?
As AI technologies advance, there are concerns about accountability, bias in algorithms, and the potential for misuse in military operations. - Can deep learning be integrated with existing military technologies?
Yes, deep learning can be integrated with various technologies, including robotics and IoT devices, to enhance operational efficiency and effectiveness.
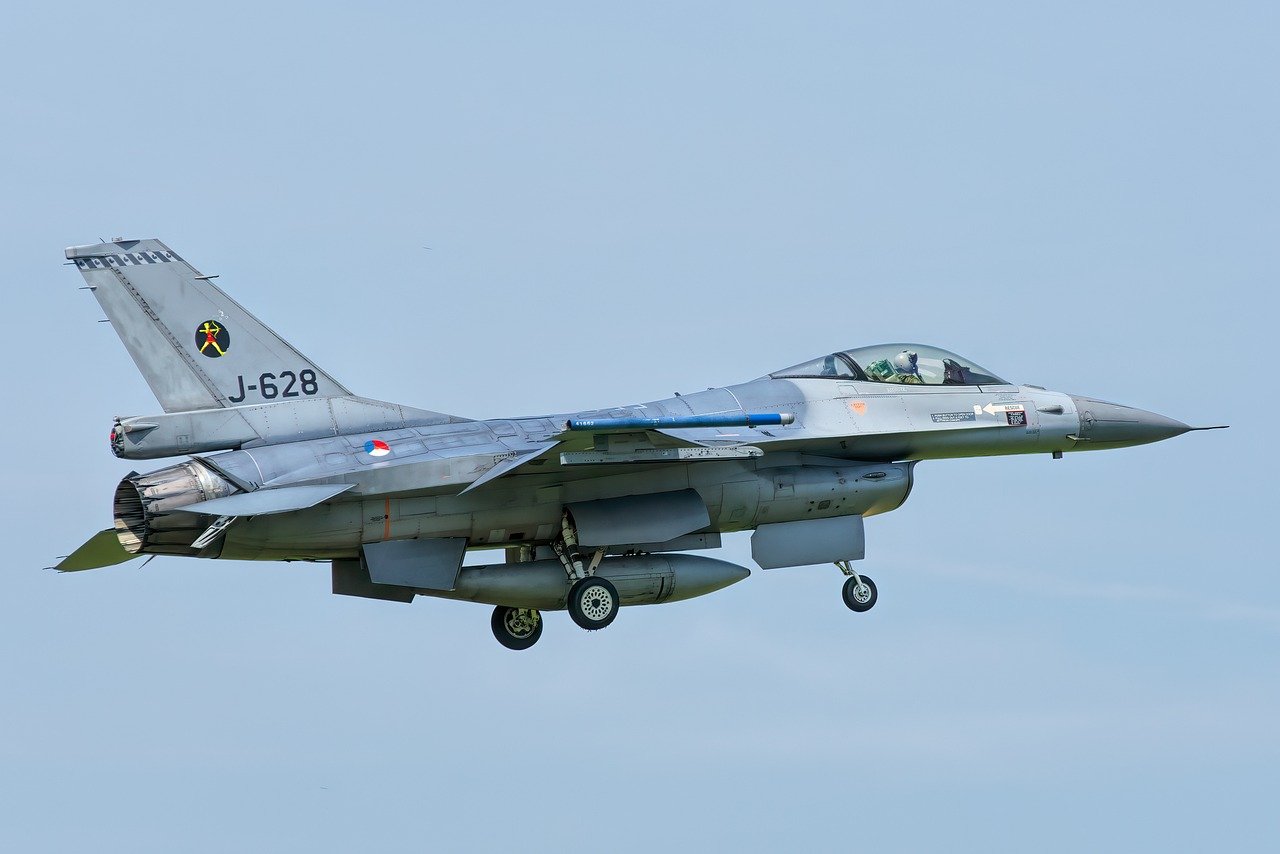
Ethical Considerations
As we delve deeper into the realm of deep learning and its applications in military image recognition, the conversation inevitably shifts towards the ethical implications of these technologies. With great power comes great responsibility, and the military's adoption of advanced AI systems poses significant moral questions that cannot be overlooked. For instance, how do we ensure that these systems are used responsibly and do not infringe upon individual rights?
One of the primary concerns revolves around accountability. When a deep learning system makes a decision—such as identifying a target or classifying a potential threat—who is held responsible if that decision leads to unintended consequences? This question is particularly poignant in scenarios where the technology could potentially lead to loss of life or collateral damage. Establishing clear lines of accountability is crucial to mitigate risks and ensure that human oversight remains paramount.
Another critical aspect is algorithmic bias. Deep learning systems are only as good as the data they are trained on. If the training datasets contain biases—whether racial, socio-economic, or geographical—these biases can be amplified in real-world applications. This could lead to discriminatory practices in military operations, where certain groups may be unfairly targeted based on flawed data. To combat this, it is essential to implement rigorous standards for data collection and processing, ensuring that the datasets used are as diverse and representative as possible.
Furthermore, the use of deep learning in military contexts raises questions about privacy. Surveillance systems equipped with advanced image recognition capabilities can monitor vast areas and track individuals without their knowledge. This capability, while enhancing security, also poses a risk of infringing on civil liberties. Striking a balance between national security and individual privacy rights is a delicate task that requires careful consideration and transparent policies.
In light of these concerns, it is vital for military organizations to develop comprehensive ethical guidelines governing the use of AI technologies. Such guidelines should address the following key areas:
- Transparency: Ensure that the decision-making processes of AI systems are understandable and can be audited.
- Human Oversight: Maintain human control over critical decisions, especially those involving life and death.
- Bias Mitigation: Actively work to identify and reduce biases in training datasets and algorithms.
- Privacy Protection: Implement measures to safeguard individual privacy rights while utilizing surveillance technologies.
In conclusion, while deep learning presents exciting opportunities for enhancing military image recognition and operational efficiency, it also necessitates a thorough examination of the ethical landscape. By proactively addressing these ethical considerations, the military can harness the power of AI responsibly, ensuring that technological advancements do not come at the cost of fundamental human values.
Q1: What are the main ethical concerns associated with deep learning in military applications?
A1: The main ethical concerns include accountability for decisions made by AI systems, algorithmic bias that can lead to discrimination, and privacy issues arising from surveillance capabilities.
Q2: How can military organizations ensure the responsible use of deep learning technologies?
A2: By developing comprehensive ethical guidelines that emphasize transparency, human oversight, bias mitigation, and privacy protection.
Q3: What role does accountability play in military AI systems?
A3: Accountability is crucial to ensure that there are clear lines of responsibility for decisions made by AI, especially in high-stakes situations where outcomes can affect lives.
Q4: Can algorithmic bias be eliminated entirely?
A4: While it may be challenging to eliminate bias completely, organizations can work to minimize it by using diverse and representative training datasets and continuously monitoring AI performance.
Frequently Asked Questions
- What is deep learning and how is it used in military image recognition?
Deep learning is a subset of artificial intelligence that uses neural networks to analyze vast amounts of data. In military image recognition, it processes complex image data to identify objects, threats, and patterns, enhancing situational awareness and operational efficiency.
- What are some specific applications of deep learning in military operations?
Deep learning is applied in various military contexts, including surveillance, target identification, and reconnaissance. These applications improve decision-making by providing real-time insights and enhancing the accuracy of threat assessments.
- How does deep learning improve surveillance and monitoring in the military?
Deep learning enhances surveillance systems by enabling real-time monitoring of extensive areas. This technology improves situational awareness and allows for quicker threat detection, which is crucial during military operations.
- What is automated threat detection, and how does it work?
Automated threat detection systems use deep learning algorithms to analyze images for potential threats. They identify anomalies and suspicious activities by recognizing patterns in data, thereby increasing military safety and response times.
- What are data fusion techniques in the context of military image recognition?
Data fusion techniques involve combining information from various sources to enhance image recognition accuracy. Deep learning facilitates this integration, allowing military intelligence to utilize diverse data types for more informed decision-making.
- What challenges does the military face when implementing deep learning?
Implementing deep learning in military image recognition presents challenges such as data quality issues, algorithm bias, and the necessity for robust training datasets. Addressing these challenges is essential for effective deployment.
- What does the future hold for deep learning in military image recognition?
The future of deep learning in military image recognition is promising, with emerging trends and advancements expected to shape military strategies. Integration with technologies like drones and robotics is likely to enhance capabilities further.
- How can deep learning integrate with other technologies in the military?
Deep learning can be integrated with technologies such as drones and robotics to create innovative solutions in image recognition. This synergy can lead to enhanced operational effectiveness and more efficient military strategies.
- What are the ethical considerations surrounding the use of deep learning in military applications?
As deep learning technologies advance, ethical considerations become increasingly important. The military must establish guidelines for responsible use to address moral implications and ensure that AI technologies are used judiciously.