The Role of Data Analytics in Counter-Terrorism Operations
In today's world, where the threat of terrorism looms large, the need for effective counter-terrorism measures has never been more critical. Data analytics has emerged as a powerful tool in the fight against terrorism, enabling agencies to sift through vast amounts of information to identify patterns, predict threats, and ultimately save lives. By leveraging advanced analytical techniques, law enforcement and intelligence agencies can transform raw data into actionable insights that enhance their operational capabilities. This article explores the vital role that data analytics plays in enhancing counter-terrorism efforts, covering various methodologies, technologies, and case studies that illustrate its effectiveness in preventing threats.
At its core, data analytics involves the systematic examination of raw data to uncover meaningful patterns and insights. Imagine trying to find a needle in a haystack; that's essentially what counter-terrorism agencies face when searching for potential threats among mountains of data. The significance of data analytics extends beyond just national security; it permeates various fields, including finance, healthcare, and marketing. However, its application in counter-terrorism is particularly crucial, as it helps agencies make sense of complex data environments and respond swiftly to emerging threats.
Several key technologies drive the field of data analytics, making it a game-changer in counter-terrorism strategies. These include machine learning, artificial intelligence, and big data frameworks. Each of these technologies plays a critical role in processing and analyzing data at unprecedented speeds. For instance, machine learning algorithms can analyze historical patterns in terrorist activities, while AI can assist in automating data processing tasks. Big data frameworks allow agencies to manage and analyze vast datasets, making it easier to detect anomalies that could indicate terrorist activities.
Machine learning is rapidly becoming a cornerstone of counter-terrorism efforts. By employing sophisticated algorithms, agencies can analyze vast datasets to identify potential threats. For example, machine learning can be used to monitor social media platforms for signs of radicalization or to analyze travel patterns that may indicate suspicious behavior. The ability to process and learn from data in real time allows agencies to stay one step ahead of potential threats.
One of the most powerful applications of data analytics in counter-terrorism is predictive analytics. This technique involves forecasting potential terrorist activities based on historical data. By developing predictive models, agencies can identify potential hotspots for terrorist activity and allocate resources more effectively. For instance, if data shows a spike in suspicious online activity in a particular region, law enforcement can increase their presence there to deter potential attacks.
In the fast-paced world of counter-terrorism, real-time data processing is essential. The ability to analyze information as it comes in can mean the difference between preventing an attack and responding to it after the fact. Agencies can utilize real-time analytics to monitor events as they unfold, enabling them to make informed decisions quickly. This immediacy is crucial, especially in high-stakes situations where every second counts.
Counter-terrorism efforts rely on a diverse array of data sources. These include:
- Social Media: Analyzing posts and interactions can reveal radicalization trends.
- Surveillance Footage: Video data can help identify suspects and their movements.
- Public Records: Information from various databases can assist in background checks.
By tapping into these varied sources, agencies can build a comprehensive picture of potential threats and act accordingly.
Despite its numerous advantages, data analytics in counter-terrorism faces significant challenges. Issues such as data privacy concerns, information overload, and the need for skilled analysts can hinder effective implementation. Agencies must navigate these obstacles carefully to ensure that they can leverage data analytics without infringing on individual rights.
The use of data analytics raises important ethical questions regarding privacy and civil liberties. Striking a balance between security measures and individual rights is paramount. Agencies must ensure that their data collection practices do not violate privacy laws or public trust. This delicate balance is essential for maintaining societal support for counter-terrorism initiatives.
Another critical challenge is ensuring data quality and integrity. Poor-quality data can lead to inaccurate analyses, resulting in misguided decisions that could have serious consequences. Agencies must implement rigorous data validation processes to maintain the integrity of their datasets. This ensures that the insights derived from data analytics are reliable and actionable.
Real-world examples of data analytics in counter-terrorism operations highlight its effectiveness. One notable case involved the use of predictive analytics to thwart an impending attack. By analyzing historical data and monitoring online chatter, agencies were able to identify a group planning an attack and intervene before it could take place. This successful intervention not only saved lives but also demonstrated the power of data analytics in action.
Specific instances where data analytics led to successful interventions provide valuable insights into its efficacy. For example, in a recent case, agencies utilized machine learning algorithms to analyze travel data, leading to the identification of a suspect before they could execute their plan. The methodologies used in these cases often involve a combination of predictive modeling and real-time monitoring, showcasing the multifaceted approach required in modern counter-terrorism efforts.
Analyzing past operations reveals key lessons learned from the application of data analytics in counter-terrorism. These insights can inform future strategies, emphasizing the importance of continuous improvement and adaptation. Agencies must remain flexible and willing to evolve their analytical techniques to stay ahead of emerging threats.
- How does data analytics improve counter-terrorism efforts?
Data analytics helps agencies identify patterns and predict potential threats, allowing for timely interventions. - What technologies are essential for data analytics in counter-terrorism?
Key technologies include machine learning, artificial intelligence, and big data frameworks. - What are the ethical concerns surrounding data analytics?
Concerns include privacy violations and the need to balance security measures with civil liberties. - How do agencies ensure data quality?
Agencies implement rigorous validation processes to maintain data integrity and reliability.
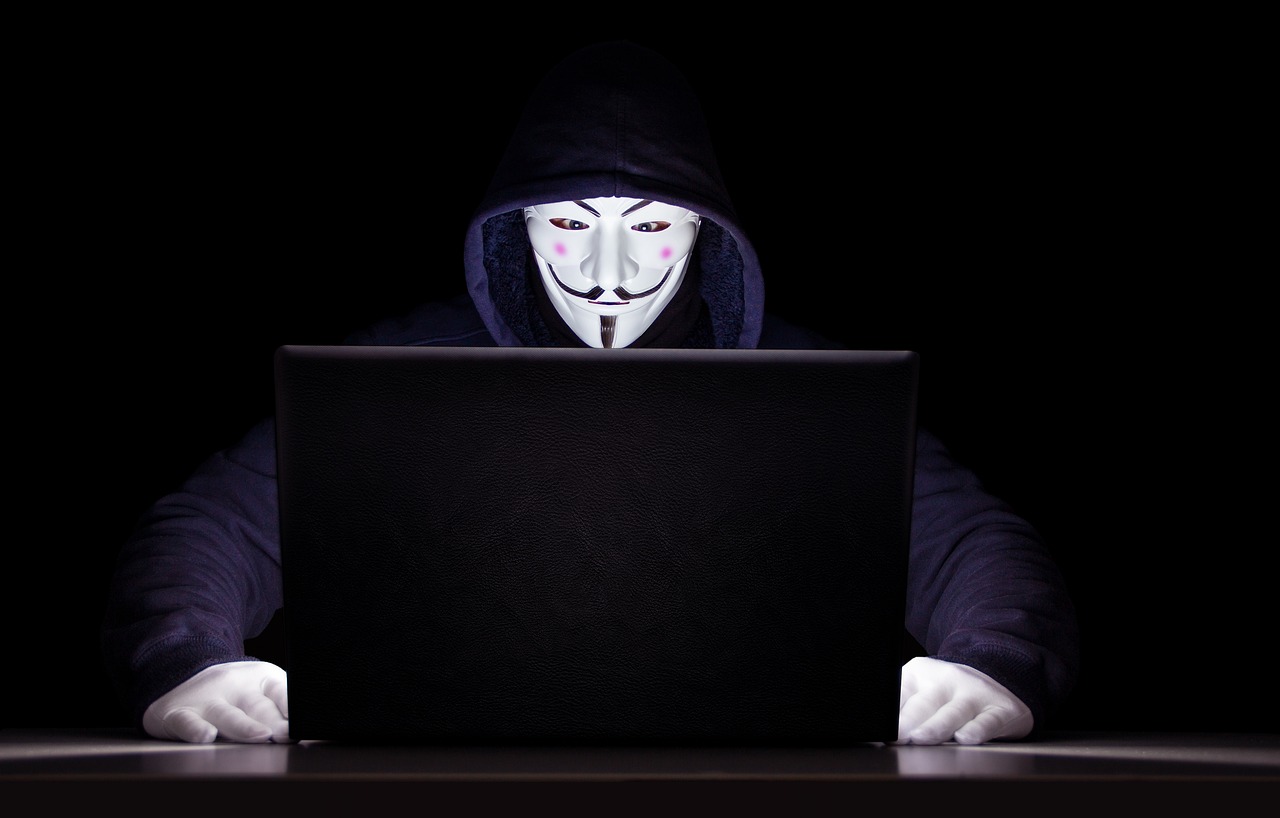
Understanding Data Analytics
Data analytics is more than just a buzzword; it's a powerful tool that transforms raw data into actionable insights. At its core, data analytics involves systematically examining data sets to uncover patterns, correlations, and trends that can inform decision-making across various fields. In the realm of national security and counter-terrorism, the significance of data analytics cannot be overstated. Just imagine trying to find a needle in a haystack—data analytics helps you not only find that needle but also understand how it got there and what it means for your safety.
In practical terms, data analytics combines statistical analysis, machine learning, and data mining techniques to process vast amounts of information. This is crucial in counter-terrorism, where the ability to quickly analyze data can mean the difference between thwarting an attack and facing dire consequences. By leveraging advanced analytics, agencies can sift through mountains of data—from social media posts to surveillance footage—allowing them to identify potential threats before they escalate.
Let’s break down the fundamental concepts of data analytics and why they’re essential in counter-terrorism:
- Descriptive Analytics: This involves summarizing historical data to understand what has happened in the past. For instance, analyzing past terrorist attacks can help identify common patterns and behaviors.
- Diagnostic Analytics: This goes a step further by explaining why something happened. It helps agencies understand the factors that led to a specific event, such as a terrorist attack.
- Predictive Analytics: This is where the magic happens! Predictive models use historical data to forecast future outcomes. In counter-terrorism, this means predicting potential attacks based on trends and patterns.
- Prescriptive Analytics: This type of analytics recommends actions based on data insights. It’s about not just knowing what could happen but also determining the best course of action to prevent it.
Moreover, the integration of data analytics into counter-terrorism strategies enables agencies to respond more effectively to threats. For example, through the analysis of social media data, agencies can detect early signs of radicalization or planned attacks, allowing for timely intervention. The ability to process and analyze data in real-time is a game-changer, providing law enforcement and intelligence agencies with the tools they need to stay one step ahead of potential threats.
However, it’s important to remember that data analytics is not a silver bullet. While it provides invaluable insights, it must be used in conjunction with human expertise and intuition. After all, data can only tell part of the story. The human element—understanding context, motivations, and cultural nuances—remains crucial in interpreting data accurately. In the world of counter-terrorism, where the stakes are incredibly high, a balanced approach that combines data analytics with human judgment is essential.
In summary, understanding data analytics is vital for enhancing counter-terrorism efforts. It empowers agencies to make informed decisions, predict potential threats, and ultimately protect communities. As technology continues to evolve, the role of data analytics in national security will only become more pronounced, making it an indispensable tool in the fight against terrorism.
- What is data analytics? Data analytics is the process of examining data sets to uncover patterns and insights that can inform decision-making.
- How does data analytics help in counter-terrorism? It allows agencies to analyze vast amounts of data quickly, identify potential threats, and make informed decisions to prevent attacks.
- What are the different types of data analytics? The main types are descriptive, diagnostic, predictive, and prescriptive analytics.
- Is data analytics foolproof? No, while it provides valuable insights, it should be used alongside human expertise for accurate interpretation.
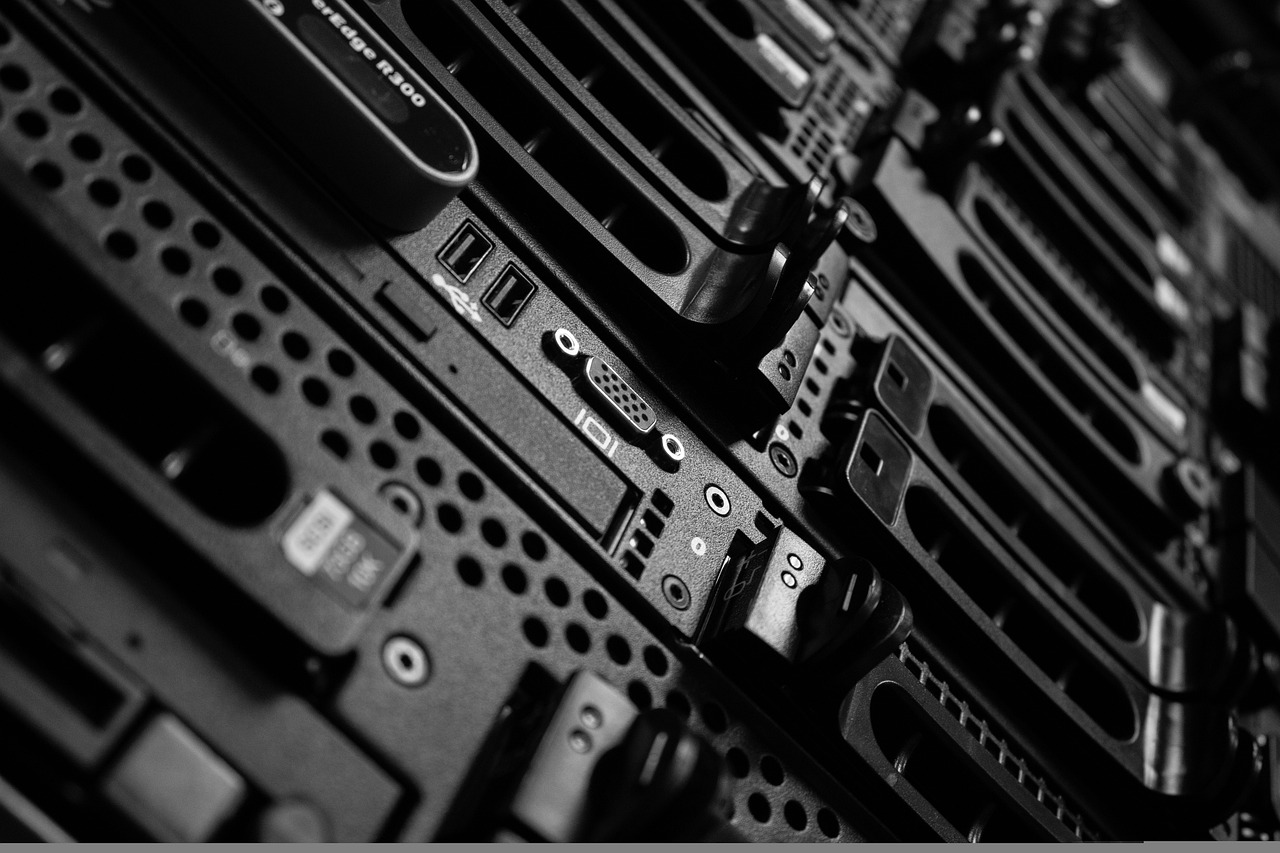
Key Technologies in Data Analytics
In the rapidly evolving landscape of counter-terrorism, data analytics serves as a cornerstone for developing effective strategies. At the heart of this transformation are several key technologies that enable analysts to sift through mountains of data, uncover patterns, and ultimately make informed decisions that can save lives. Machine learning, artificial intelligence, and big data frameworks are the heavyweights in this arena, each playing a unique role in enhancing our ability to predict and prevent terrorist activities.
Machine learning is a game changer. Imagine teaching a computer to recognize patterns in data just like you would train a dog to fetch a ball. By feeding algorithms vast amounts of historical data, they learn to identify anomalies that could signify potential threats. This technology is particularly adept at processing unstructured data, such as text and images, which are often abundant in surveillance and social media feeds. For instance, machine learning models can analyze social media posts for signs of radicalization or detect unusual patterns in communication among known individuals.
When we dive deeper into machine learning applications, we see its utility in various scenarios. For example, algorithms can be employed to analyze flight data, scrutinizing passenger behaviors and travel patterns. By comparing these patterns against historical data, authorities can flag suspicious activities that may require further investigation.
Another significant technology is artificial intelligence (AI). AI enhances data analytics by simulating human intelligence processes. It can automate the analysis of data streams, allowing for quicker responses to potential threats. Think of AI as a virtual assistant that never tires and can analyze data faster than any human ever could. This capability is essential in situations where every second counts, such as in the case of a potential terrorist attack.
Moreover, big data frameworks provide the infrastructure necessary to handle the enormous volumes of data generated today. Technologies like Hadoop and Spark allow for distributed processing of large datasets, making it possible to analyze data in real-time. This capability is crucial for counter-terrorism efforts, as it enables agencies to act swiftly based on the latest available information. For instance, if a new threat emerges online, agencies can quickly gather and analyze relevant data to assess the situation.
In summary, the interplay of these technologies creates a robust ecosystem for data analytics in counter-terrorism. By leveraging machine learning, artificial intelligence, and big data frameworks, agencies can enhance their operational efficiency and effectiveness. The result? A more proactive approach to identifying and neutralizing threats before they escalate.
- What is data analytics in counter-terrorism?
Data analytics in counter-terrorism involves analyzing large datasets to identify patterns and insights that can help prevent terrorist activities.
- How does machine learning contribute to counter-terrorism?
Machine learning algorithms can analyze vast amounts of data to identify potential threats and anomalies, allowing for quicker intervention.
- What role does artificial intelligence play in data analytics?
AI automates the analysis of data, enabling faster processing and decision-making, which is critical in urgent situations.
- Why is big data important in counter-terrorism?
Big data frameworks allow for the processing and analysis of large volumes of information, which is essential for real-time threat assessment.
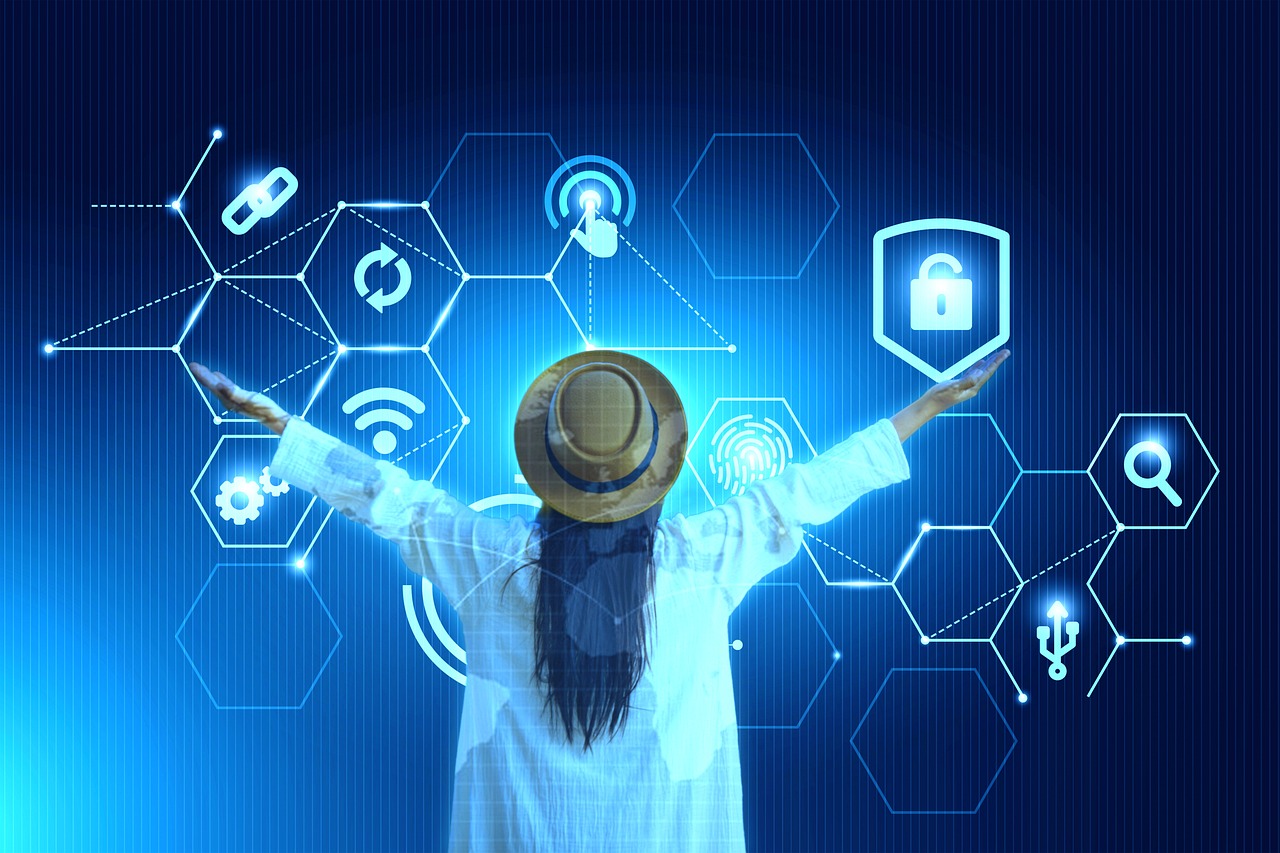
Machine Learning Applications
In the realm of counter-terrorism, machine learning has emerged as a game-changer, providing agencies with the ability to sift through enormous datasets and identify potential threats with unprecedented accuracy. Imagine trying to find a needle in a haystack; now imagine that haystack is made up of billions of data points from various sources. This is where machine learning shines, using complex algorithms to detect patterns that human analysts might overlook. By analyzing historical data, social media trends, and even communication patterns, machine learning models can predict where and when potential threats may arise.
One of the most compelling applications of machine learning in counter-terrorism is in the area of threat detection. For instance, algorithms can be trained to recognize the digital footprints of known terrorist organizations by analyzing their communication habits, online behavior, and even financial transactions. By continuously learning from new data, these models become increasingly adept at flagging suspicious activities, ensuring that law enforcement agencies can act swiftly to prevent potential attacks.
Moreover, machine learning is not just about identifying threats; it also plays a crucial role in resource allocation. By analyzing data on past incidents, agencies can optimize their deployment of personnel and resources. For example, if a specific area has seen a spike in suspicious activity, machine learning algorithms can help predict where law enforcement presence is most needed, thereby enhancing operational efficiency.
To illustrate the effectiveness of machine learning in counter-terrorism, consider the following table that outlines some common applications:
Application | Description |
---|---|
Social Media Monitoring | Analyzing social media posts to identify potential threats and extremist sentiments. |
Behavioral Analysis | Using data from previous incidents to identify patterns in terrorist behavior. |
Financial Transaction Monitoring | Tracking unusual financial activities that may indicate funding for terrorist operations. |
Surveillance Data Analysis | Processing video feeds to detect suspicious activities in real-time. |
In addition to these applications, machine learning also supports predictive analytics. This involves using historical data to forecast future events, allowing agencies to anticipate and mitigate threats before they materialize. For example, if a particular group has a history of activity during specific times of the year, machine learning models can alert authorities to increase surveillance during those periods.
In summary, the applications of machine learning in counter-terrorism are vast and varied, transforming how agencies approach threat detection and prevention. As technology continues to evolve, we can expect machine learning to play an even more pivotal role in safeguarding national security.
- What is machine learning? - Machine learning is a subset of artificial intelligence that enables computers to learn from data and make predictions or decisions without being explicitly programmed.
- How does machine learning help in counter-terrorism? - It helps by analyzing vast amounts of data to identify patterns and predict potential threats, enabling timely intervention.
- Are there any ethical concerns with using machine learning in counter-terrorism? - Yes, there are concerns regarding data privacy and the potential for bias in algorithms, which can impact civil liberties.
- Can machine learning replace human analysts in counter-terrorism? - While machine learning enhances the capabilities of human analysts, it is not a replacement; human judgment is still crucial in interpreting data.
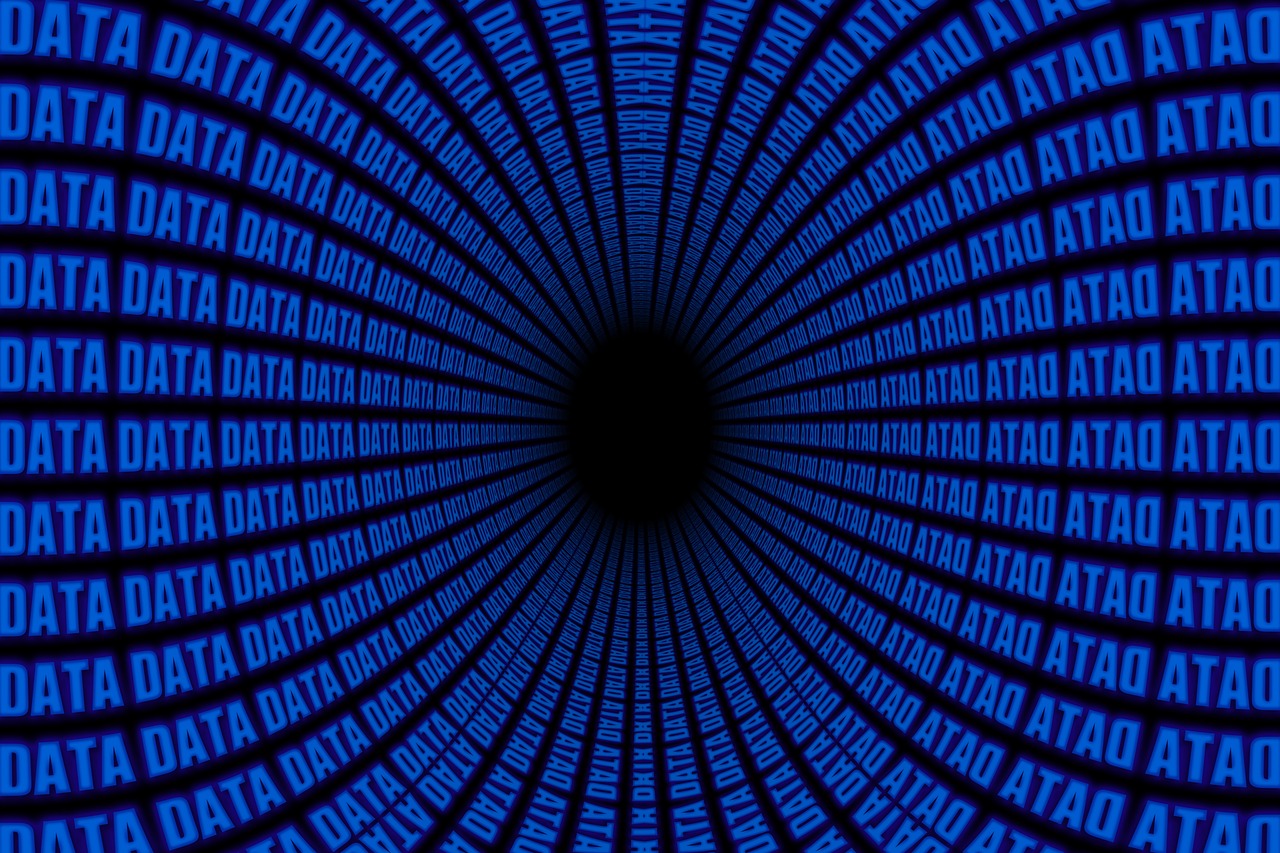
Predictive Analytics
Predictive analytics is like having a crystal ball that helps counter-terrorism agencies foresee potential threats before they manifest. By leveraging historical data, agencies can create models that not only identify patterns but also predict future behaviors and activities. Imagine being able to anticipate a storm before it hits; that’s the power of predictive analytics in the realm of national security. These models analyze vast amounts of data, ranging from social media activity to financial transactions, and distill it into actionable insights that can guide decision-making.
At its core, predictive analytics employs complex algorithms and statistical techniques to sift through data and identify trends that may indicate an impending terrorist act. For instance, if a specific group shows a pattern of online chatter about a particular event, predictive models can flag this activity for further investigation. This proactive approach is crucial because it allows agencies to allocate resources effectively and respond swiftly to potential threats.
To better illustrate how predictive analytics works in counter-terrorism, consider the following components involved in the process:
Component | Description |
---|---|
Data Collection | Gathering data from various sources such as social media, public records, and surveillance. |
Data Processing | Cleaning and organizing the data to make it suitable for analysis. |
Model Development | Creating statistical models that can identify patterns and predict future events. |
Analysis and Interpretation | Examining model outputs to draw conclusions and make informed decisions. |
Action Implementation | Taking proactive measures based on insights gained from the analysis. |
The effectiveness of predictive analytics in counter-terrorism is evident in various real-world applications. For example, some agencies have successfully used these models to identify potential hotspots for terrorist recruitment or activity. By analyzing social media trends, they can pinpoint areas where extremist ideologies are gaining traction and intervene before these ideas can take root.
However, it’s essential to recognize that predictive analytics is not foolproof. While it provides valuable insights, it also carries the risk of false positives, where innocent behaviors may be misinterpreted as suspicious. This underscores the need for a balanced approach that combines predictive analytics with human intelligence and oversight. Ultimately, the goal is to enhance national security while respecting civil liberties and ensuring that the data is used ethically and responsibly.
In conclusion, predictive analytics serves as a powerful tool in the arsenal of counter-terrorism strategies. By anticipating threats and enabling timely interventions, it helps create a safer environment for everyone. As technology continues to evolve, the potential for predictive analytics to further enhance counter-terrorism efforts is immense, paving the way for a more secure future.
- What is predictive analytics? Predictive analytics involves using historical data and statistical algorithms to predict future events or behaviors.
- How does predictive analytics help in counter-terrorism? It helps by identifying potential threats and enabling agencies to take proactive measures to prevent terrorist activities.
- Are there risks associated with predictive analytics? Yes, there are risks such as false positives and ethical concerns regarding privacy and civil liberties.
- What types of data are used in predictive analytics? Data sources can include social media, financial records, public databases, and surveillance footage.
- Can predictive analytics guarantee safety? While it enhances threat detection, it cannot guarantee complete safety; it should be used alongside other intelligence methods.
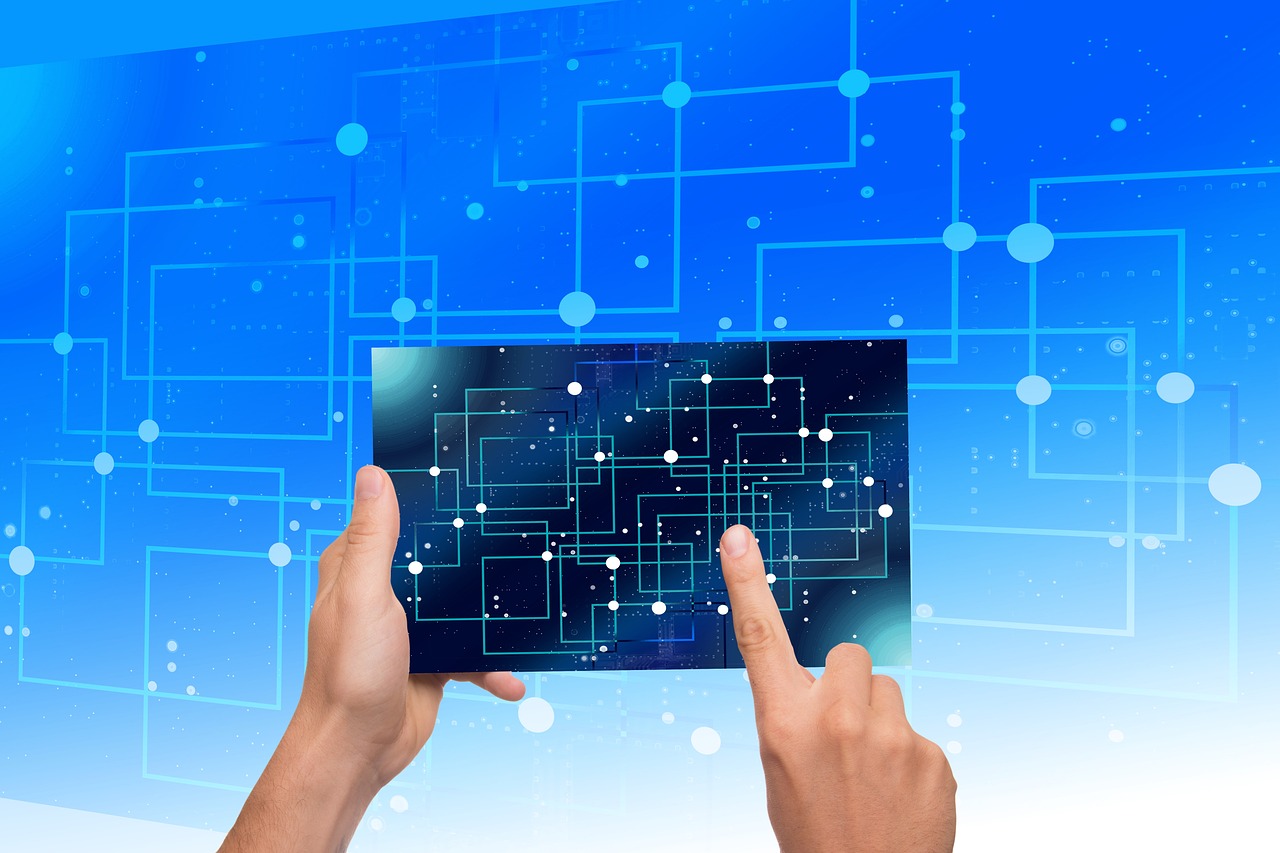
Real-time Data Processing
In the fast-paced world of counter-terrorism, is akin to having a superhero on your side. Imagine being able to analyze information as it streams in, catching potential threats before they escalate. This capability is not just a luxury; it’s a necessity. With data coming from various sources—social media, surveillance systems, and even public records—agencies must sift through this overwhelming amount of information instantly to identify and neutralize threats.
Real-time data processing allows intelligence agencies to act on information as it becomes available, which is crucial in thwarting terrorist plots. For instance, if a suspicious activity is detected on social media, the ability to analyze that data in real-time can lead to immediate action, potentially preventing an attack. This immediacy can mean the difference between safety and disaster.
To achieve effective real-time data processing, agencies utilize sophisticated technologies that can handle vast amounts of data quickly. These technologies often include:
- Stream Processing Frameworks: These frameworks allow for the continuous input and analysis of data streams, enabling agencies to monitor activities in real-time.
- Automated Alert Systems: These systems notify analysts of potential threats as soon as they are detected, ensuring that no critical information slips through the cracks.
- Data Visualization Tools: By presenting data in an easily digestible format, these tools help analysts quickly identify patterns and anomalies.
However, real-time data processing is not without its challenges. The sheer volume of data can lead to information overload, where analysts may struggle to discern actionable intelligence from noise. To counteract this, agencies often implement machine learning algorithms that filter and prioritize data, ensuring that only the most relevant information reaches the analysts.
Moreover, the integration of Artificial Intelligence (AI) plays a pivotal role in enhancing real-time data processing capabilities. AI can quickly identify patterns that might go unnoticed by human analysts, providing insights that are not only timely but also predictive. This synergy between human intelligence and AI-driven analytics creates a robust framework for counter-terrorism operations.
In conclusion, real-time data processing is a game-changer in the realm of counter-terrorism. By enabling agencies to respond promptly to emerging threats, it significantly enhances national security efforts. As technology continues to evolve, the effectiveness of real-time data processing will undoubtedly improve, paving the way for a safer world.
- What is real-time data processing in counter-terrorism?
Real-time data processing refers to the ability to analyze and act on data as it is received, allowing for immediate responses to potential threats. - How does machine learning enhance real-time data processing?
Machine learning algorithms can quickly filter through large datasets to identify relevant information, helping analysts focus on actionable intelligence. - What are some challenges of real-time data processing?
Challenges include information overload, ensuring data accuracy, and the need for skilled personnel to interpret the data effectively. - Why is timely data important in counter-terrorism?
Timely data allows agencies to prevent terrorist activities before they occur, thereby saving lives and maintaining public safety.
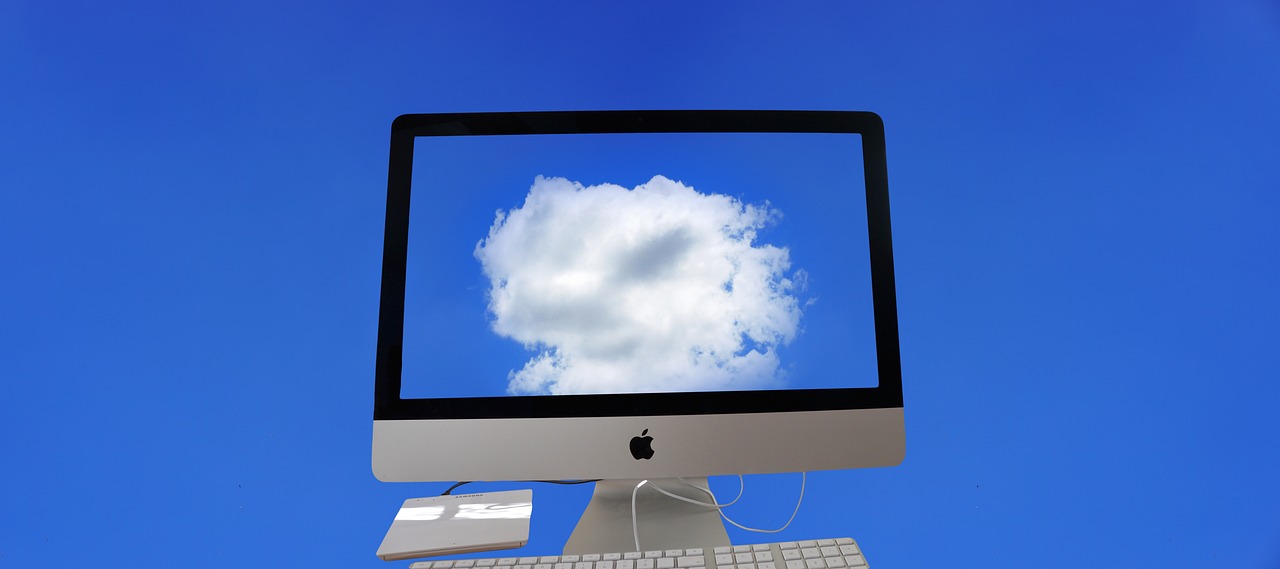
Data Sources for Counter-Terrorism
In the ever-evolving landscape of counter-terrorism, the ability to gather and analyze data from a myriad of sources is crucial. The effectiveness of counter-terrorism operations heavily relies on the integration of diverse data streams. Think of it like assembling a jigsaw puzzle; each piece of data contributes to a clearer picture of potential threats. One of the primary sources of data comes from social media platforms. With billions of users sharing their thoughts, activities, and even intentions online, social media serves as a goldmine for intelligence agencies. By employing sophisticated algorithms, analysts can sift through vast amounts of posts, identifying patterns or anomalies that may indicate suspicious behavior.
Another significant source is surveillance footage. With the proliferation of CCTV cameras in urban areas, authorities can monitor public spaces in real-time. This visual data can be analyzed to track individuals or groups of interest, providing insights into their movements and behaviors. However, the challenge lies in managing and analyzing this data efficiently, especially when dealing with thousands of hours of footage.
Additionally, public records play a vital role in counter-terrorism efforts. These records can include everything from financial transactions to travel histories. By analyzing these documents, agencies can uncover links between individuals and organizations that may pose a threat. For instance, tracking unusual financial patterns can alert authorities to potential funding for terrorist activities.
Moreover, geospatial data is increasingly being utilized in counter-terrorism operations. Geographic Information Systems (GIS) can help map out areas of interest, allowing for better resource allocation and strategic planning. By visualizing data geographically, analysts can identify hotspots for potential terrorist activity, enabling proactive measures to be taken.
To summarize, the data sources for counter-terrorism are as diverse as the threats themselves. Here’s a quick overview of some key sources:
- Social Media: Valuable for real-time sentiment analysis and identifying potential threats.
- Surveillance Footage: Essential for tracking movements and gathering visual evidence.
- Public Records: Useful for uncovering financial and personal connections.
- Geospatial Data: Helps in mapping out potential risk areas for strategic interventions.
Ultimately, the integration of these data sources creates a comprehensive intelligence framework that enhances the ability to detect and prevent terrorist activities. However, it’s important to remember that while data is a powerful tool, it must be used responsibly and ethically to protect civil liberties.
- What role does social media play in counter-terrorism?
Social media platforms are used to monitor public sentiment and detect potential threats through user-generated content. - How is surveillance footage analyzed?
Agencies use advanced algorithms and machine learning techniques to analyze large volumes of video data for suspicious activities. - What types of public records are useful in counter-terrorism?
Financial transactions, travel records, and criminal histories are examples of public records that can help identify potential threats. - How does geospatial data assist in counter-terrorism?
Geospatial data allows analysts to visualize and map areas of interest, helping to identify hotspots for potential terrorist activities.
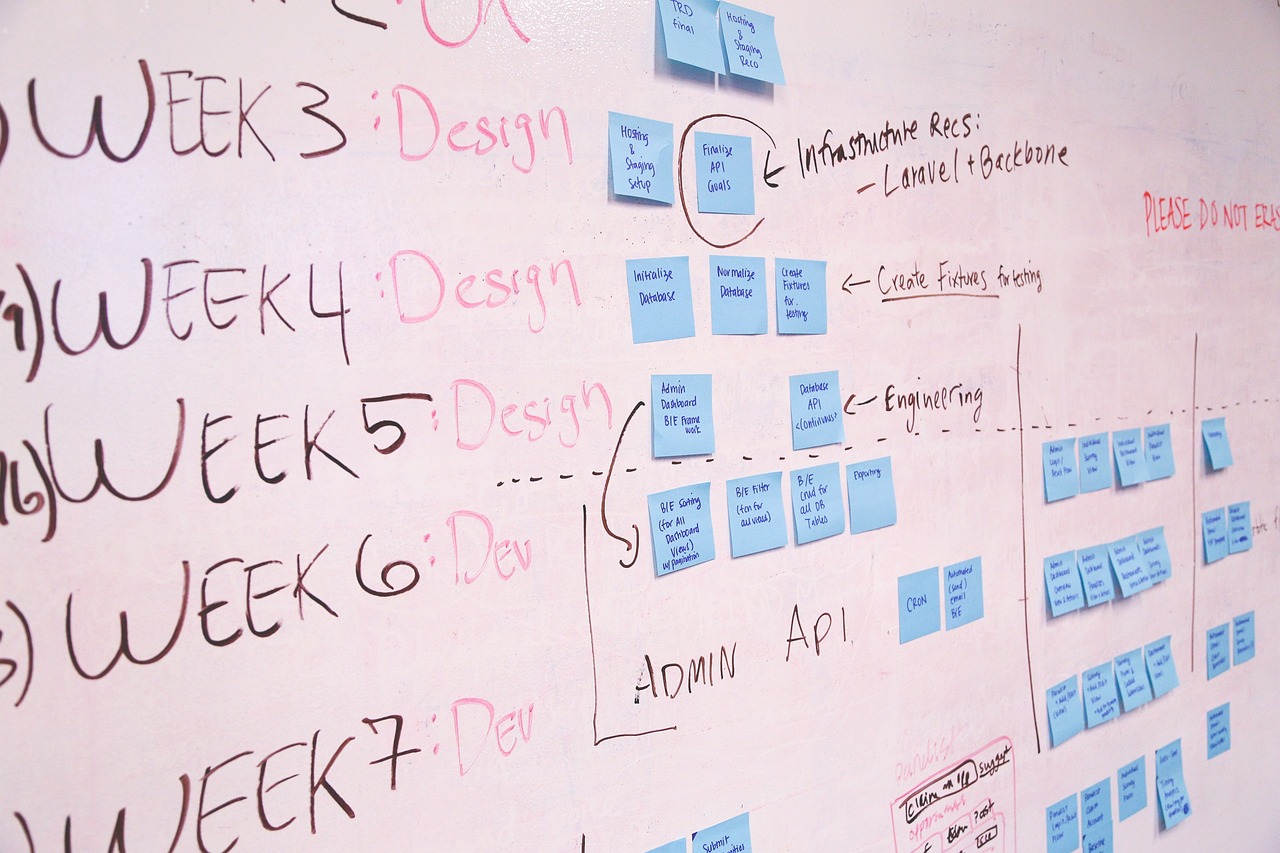
Challenges in Data Analytics
While data analytics has revolutionized the way we approach counter-terrorism, it is not without its challenges. One of the most pressing issues is data privacy concerns. As government agencies and organizations collect vast amounts of data to identify potential threats, the line between national security and individual privacy can become blurred. Citizens often worry that their personal information may be misused or that surveillance might infringe on their civil liberties. This creates a delicate balance that must be maintained to ensure that security measures do not come at the expense of fundamental rights.
Another significant challenge is information overload. In the age of big data, the sheer volume of information available can be overwhelming. Analysts are often inundated with data from multiple sources, including social media, public records, and surveillance systems. Without effective filtering and prioritization methods, it can be difficult to extract meaningful insights from the noise. This can lead to critical information being overlooked, potentially allowing threats to slip through the cracks.
Furthermore, there is a persistent need for skilled analysts who can interpret complex data sets and draw actionable conclusions. The rapid evolution of technology, particularly in fields like machine learning and artificial intelligence, necessitates continuous training and development for personnel involved in counter-terrorism efforts. Organizations may struggle to recruit and retain talent with the necessary expertise, which can hinder the effectiveness of their data analytics initiatives.
To illustrate these challenges, consider the following table that outlines some of the key obstacles faced by data analysts in the counter-terrorism domain:
Challenge | Description |
---|---|
Data Privacy Concerns | Balancing national security with individual rights and privacy. |
Information Overload | Handling vast amounts of data without losing critical insights. |
Need for Skilled Analysts | Recruiting and retaining experts who can navigate complex data. |
In addition to these challenges, there are also ethical considerations that must be taken into account. The use of data analytics in counter-terrorism raises questions about the morality of surveillance and the potential for misuse of information. How do we ensure that data is used responsibly and ethically? This is a question that agencies must grapple with as they implement data-driven strategies.
Lastly, data quality and integrity play a pivotal role in the success of any analytics effort. Poor-quality data can lead to inaccurate conclusions, misinformed decisions, and ultimately, failed interventions. Ensuring that the data being analyzed is both reliable and valid is crucial for effective counter-terrorism operations. Analysts must be diligent in their efforts to verify data sources and maintain the integrity of the information they work with.
- What are the main challenges in data analytics for counter-terrorism?
Data privacy concerns, information overload, the need for skilled analysts, ethical considerations, and data quality issues are the main challenges faced. - How does information overload affect counter-terrorism efforts?
Information overload can lead to critical data being overlooked, which may allow potential threats to go undetected. - Why is data quality important in counter-terrorism analytics?
High-quality data ensures accurate analysis and informed decision-making, which is essential for effective counter-terrorism strategies.
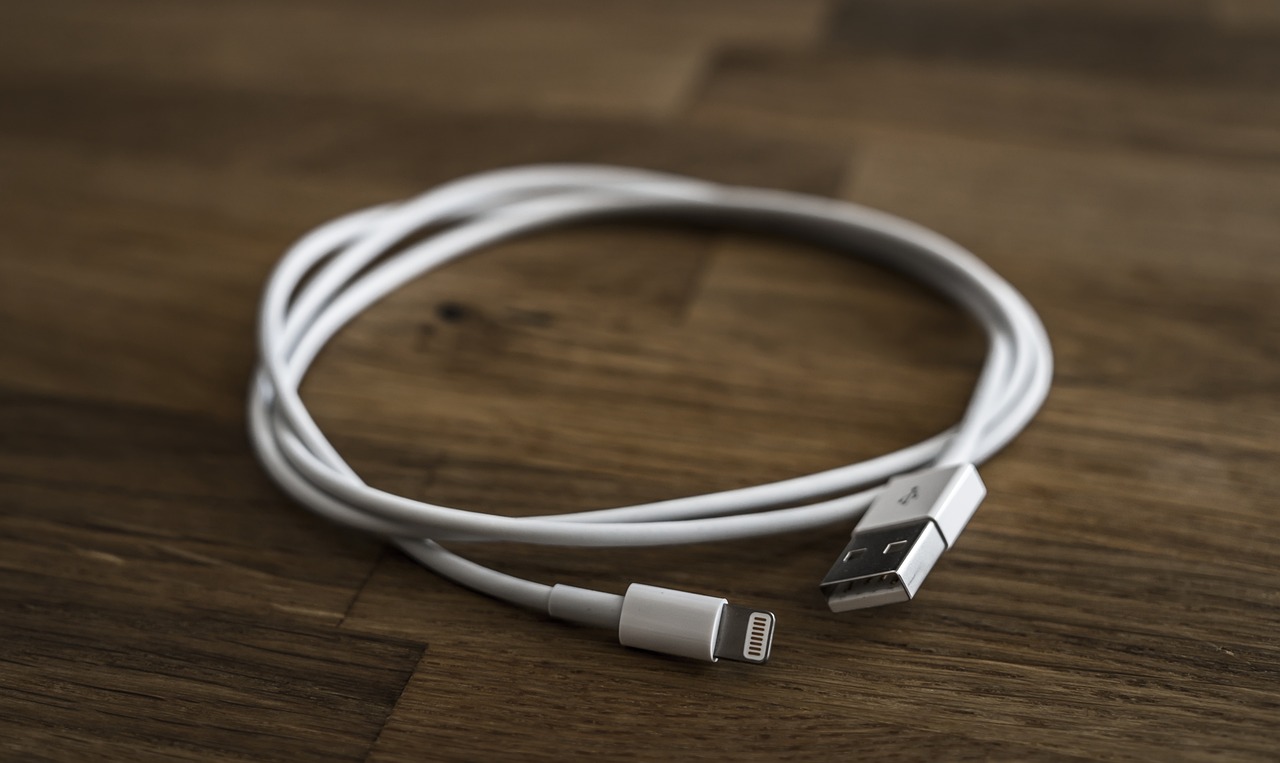
Ethical Considerations
The rise of data analytics in counter-terrorism has sparked a heated debate about the ethical implications of its use. As we delve into this complex landscape, it becomes clear that while data analytics offers powerful tools to prevent attacks, it also raises significant concerns about privacy and civil liberties. The challenge is to strike a balance between ensuring national security and protecting individual rights.
One primary ethical concern revolves around the surveillance of citizens. With the ability to analyze vast amounts of data, agencies can monitor communications, social media activity, and even movements in real-time. This level of surveillance can feel invasive to the public and may lead to a chilling effect on free speech and expression. Imagine living in a society where every tweet, every text, and every online interaction is scrutinized—would you feel free to express your thoughts? This is the crux of the ethical dilemma.
Moreover, the potential for discrimination based on data analytics cannot be overlooked. Algorithms used in profiling can inadvertently reinforce existing biases, leading to the unfair targeting of specific groups. This raises the question: how do we ensure that data-driven decisions are fair and just? The answer lies in transparency and accountability in the algorithms and models used. By making these processes open to scrutiny, we can work towards minimizing bias and ensuring that ethical standards are upheld.
Additionally, there are concerns about data security. As agencies collect and store sensitive information, the risk of data breaches increases. If personal data falls into the wrong hands, it could be misused, leading to serious repercussions for individuals. Therefore, it's crucial that robust security measures are implemented to protect this data. Agencies must be held accountable for maintaining the integrity and confidentiality of the information they collect.
To navigate these ethical waters, several principles should guide the use of data analytics in counter-terrorism:
- Transparency: Agencies should be open about how data is collected, analyzed, and used.
- Accountability: There should be mechanisms in place to hold agencies responsible for their actions.
- Public Engagement: Involving the public in discussions about data use can help build trust and understanding.
- Data Minimization: Collect only the data necessary for specific purposes, reducing the risk of misuse.
In conclusion, while data analytics is a powerful ally in the fight against terrorism, it’s essential to address the ethical considerations that come with its use. By prioritizing privacy, fairness, and security, we can harness the benefits of data analytics while safeguarding the rights of individuals. The conversation about ethics in data analytics is ongoing, and it’s one that we all need to engage in to ensure a balanced approach to national security.
- What are the main ethical concerns regarding data analytics in counter-terrorism? The primary concerns include privacy invasion, potential discrimination, and data security risks.
- How can agencies ensure fairness in data analytics? By implementing transparency, accountability, and public engagement, agencies can work to minimize bias in their analytics.
- What measures can be taken to protect sensitive data? Agencies should employ robust security protocols and practice data minimization to protect personal information.

Data Quality and Integrity
In the realm of counter-terrorism, data quality and integrity are paramount. Imagine trying to navigate through a dense fog without a clear map; that’s what it feels like for analysts working with poor-quality data. When the stakes are this high, the reliability of the information being analyzed can mean the difference between thwarting a potential attack and letting it slip through the cracks. High-quality data provides a solid foundation for effective analysis, while compromised data can lead to misguided conclusions and wasted resources.
Data quality refers to the accuracy, completeness, consistency, and reliability of the data being used. In counter-terrorism operations, this means that every piece of information—from social media posts to surveillance footage—must be scrutinized for its validity. Without rigorous checks in place, analysts may find themselves sifting through a mountain of irrelevant or erroneous data, ultimately slowing down decision-making processes. This is particularly critical when real-time responses are required to neutralize threats.
Moreover, the integrity of data is equally crucial. It involves ensuring that the data remains unaltered and trustworthy throughout its lifecycle. Any tampering or unauthorized access can lead to catastrophic consequences. For example, if a terrorist organization manages to manipulate their digital footprint, it could mislead intelligence agencies into believing they are not a threat. Hence, maintaining data integrity is not just about protection; it's about ensuring that the information used for analysis is genuine and reflective of reality.
To address these challenges, agencies often implement a multi-faceted approach that includes:
- Data Validation Techniques: These techniques help verify the authenticity of the data collected, ensuring that it meets predefined standards before it is used for analysis.
- Regular Audits: Conducting periodic audits on data sources helps identify discrepancies and rectify them before they can affect operational decisions.
- Training Analysts: Providing analysts with the necessary training to recognize and handle data quality issues is essential. This empowers them to make informed decisions based on reliable information.
In addition, agencies can employ advanced technologies to enhance data quality and integrity. For instance, utilizing blockchain technology can provide a secure and transparent way to manage data, making it nearly impossible for unauthorized changes to go unnoticed. Furthermore, machine learning algorithms can be designed to flag anomalies in data patterns, alerting analysts to potential quality issues before they escalate.
Ultimately, the importance of data quality and integrity in counter-terrorism cannot be overstated. As threats evolve and become more sophisticated, so too must the methods used to analyze the data related to these threats. By prioritizing high-quality data and maintaining its integrity, agencies can significantly improve their chances of successfully preventing terrorist activities.
- What is data quality in the context of counter-terrorism? Data quality refers to the accuracy, completeness, and reliability of data used in counter-terrorism operations, which is crucial for making informed decisions.
- Why is data integrity important? Data integrity ensures that the information remains unchanged and trustworthy, reducing the risk of misleading conclusions that could lead to security failures.
- How can agencies ensure data quality? Agencies can implement data validation techniques, conduct regular audits, and provide training to analysts to enhance data quality.
- What role does technology play in maintaining data integrity? Technologies like blockchain can secure data management, while machine learning can identify anomalies, helping to maintain data integrity.
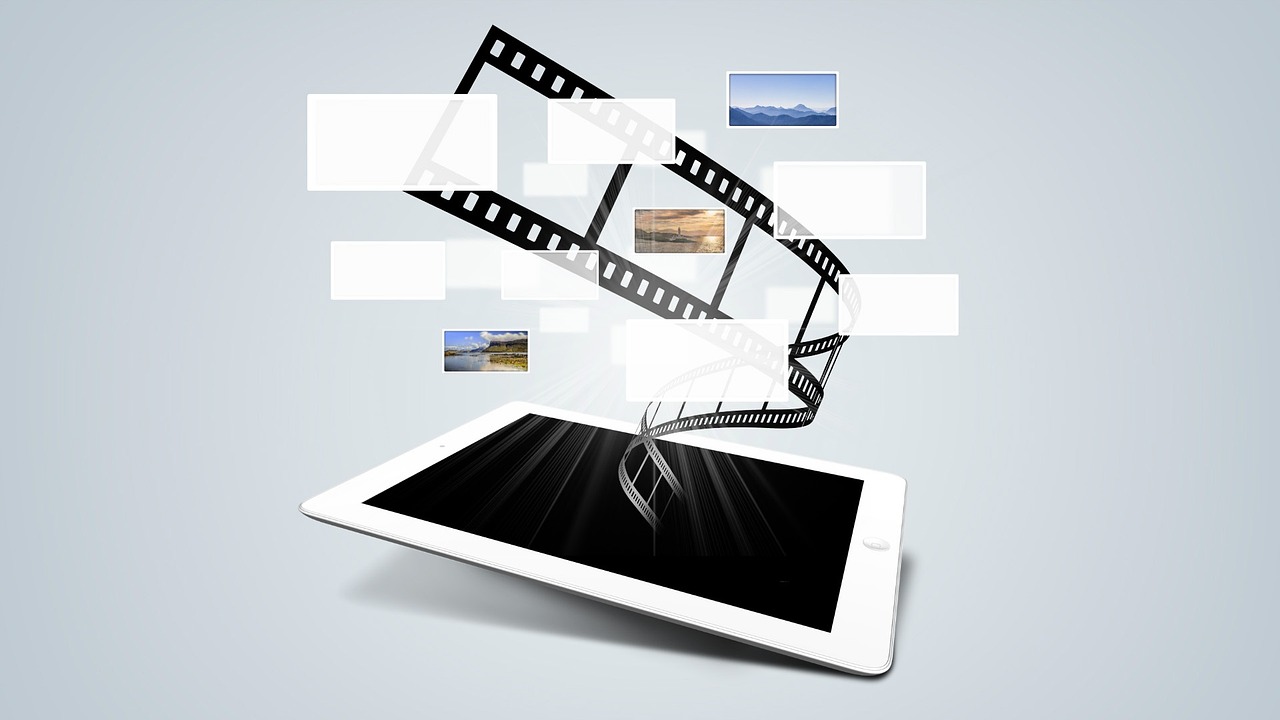
Case Studies of Data Analytics in Action
The application of data analytics in counter-terrorism has led to remarkable successes that underscore its significance. These real-world examples not only showcase the methodologies employed but also highlight the outcomes achieved in thwarting potential terrorist activities. One notable case involved the use of machine learning algorithms to analyze social media interactions and identify patterns indicative of extremist behavior. By monitoring specific keywords and phrases, analysts were able to flag suspicious accounts and track their activities in real time.
Another compelling example comes from a large-scale operation where predictive analytics was utilized to assess threats based on historical data. In this instance, a government agency developed a model that analyzed past terrorist attacks, taking into account various factors such as location, timing, and methods used. The insights gained allowed them to prioritize resource allocation in areas deemed high-risk, effectively preventing a planned attack that had been in the works for months.
Furthermore, the integration of real-time data processing has proven invaluable in counter-terrorism efforts. For instance, during a public event, law enforcement agencies utilized surveillance footage combined with crowd-sourced data to monitor potential threats. By analyzing the data as it streamed in, they were able to identify unusual behavior patterns and respond immediately, ensuring the safety of attendees. This case exemplifies how timely data can be the difference between chaos and control.
To better illustrate the impact of data analytics in these operations, consider the following table that summarizes key case studies:
Case Study | Methodology Used | Outcome |
---|---|---|
Social Media Monitoring | Machine Learning Algorithms | Identification of extremist accounts, prevention of online radicalization |
Threat Assessment | Predictive Analytics | Prevention of a planned attack through resource allocation |
Event Security | Real-Time Data Processing | Immediate identification of potential threats, enhanced public safety |
These case studies not only demonstrate the effectiveness of data analytics in counter-terrorism but also highlight the continuous evolution of technology and methodologies in this field. The lessons learned from these operations are invaluable, informing future strategies and enhancing the capabilities of security agencies worldwide.
Successful interventions in counter-terrorism often hinge on the ability to analyze data effectively. For example, a recent operation in a major city involved the collaboration of various agencies that pooled their data resources. By leveraging data from multiple sources, including public records and intelligence reports, they successfully disrupted a terrorist cell planning an attack. This intervention was not just a stroke of luck; it was the result of a well-coordinated effort to harness data analytics for proactive measures.
Moreover, the insights gained from these successful interventions lead to the development of best practices in data analysis. Agencies are now more equipped than ever to make data-driven decisions, allowing them to stay one step ahead of potential threats.
Analyzing past operations provides critical insights that can shape future counter-terrorism strategies. One of the key lessons learned is the importance of collaboration between agencies. The sharing of data and resources has proven to be a game-changer in identifying and neutralizing threats. Additionally, the necessity of ongoing training for analysts cannot be overstated. As technology continues to evolve, so too must the skills of those tasked with interpreting data.
In conclusion, the case studies of data analytics in action illustrate not only the power of technology in counter-terrorism but also the human ingenuity behind it. By continuously refining methodologies and embracing innovative technologies, security agencies can enhance their capabilities to protect the public from evolving threats.
Q1: How does data analytics help in counter-terrorism?
Data analytics helps in counter-terrorism by uncovering patterns and insights from large datasets, enabling agencies to identify potential threats and intervene proactively.
Q2: What technologies are commonly used in data analytics for counter-terrorism?
Common technologies include machine learning, artificial intelligence, and big data frameworks, which all contribute to more effective threat detection and analysis.
Q3: Are there ethical concerns associated with data analytics in counter-terrorism?
Yes, ethical concerns such as privacy and civil liberties are significant issues that need to be balanced against the need for security in counter-terrorism operations.
Q4: Can data analytics prevent terrorist attacks?
While data analytics cannot guarantee the prevention of all attacks, it significantly enhances the ability of agencies to identify and neutralize threats before they materialize.
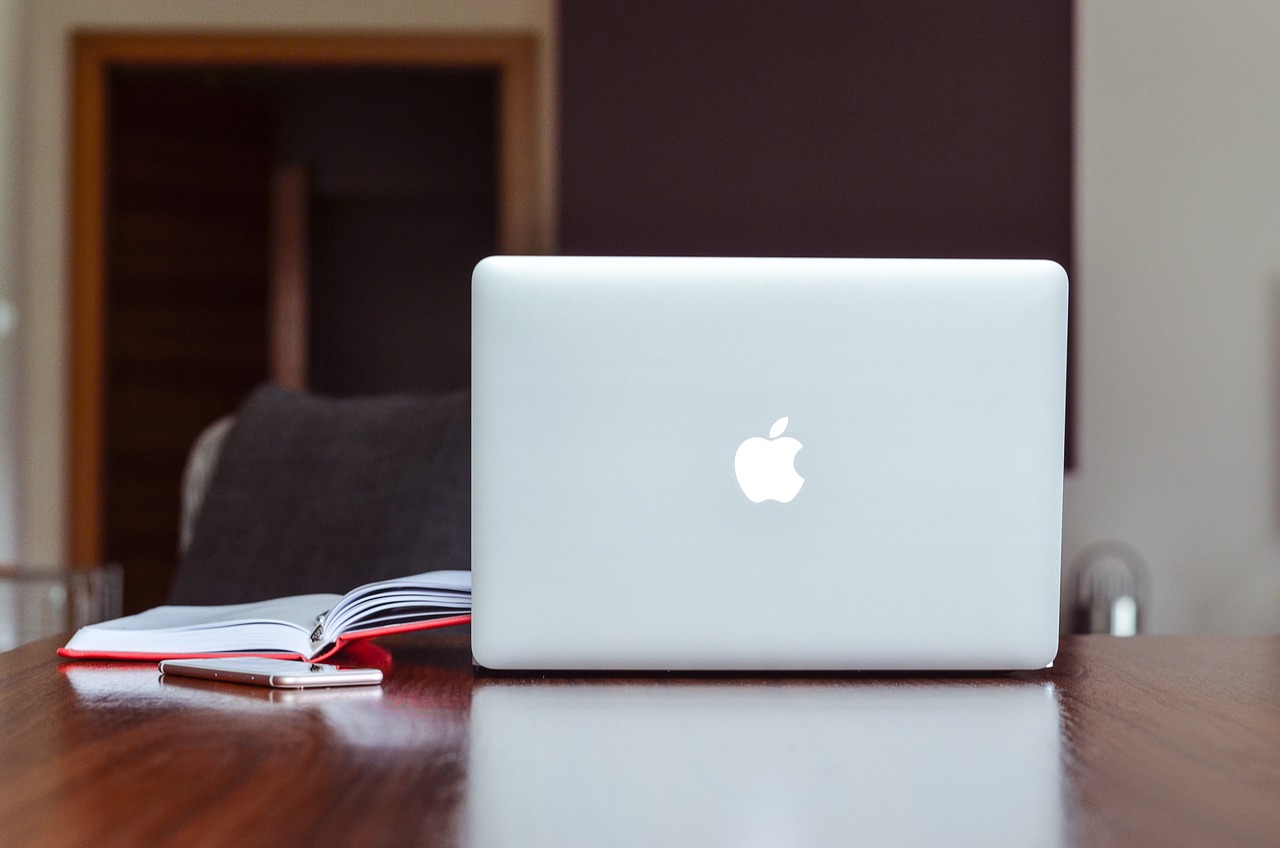
Successful Interventions
Data analytics has proven to be a powerful ally in the fight against terrorism, leading to numerous successful interventions that have saved countless lives. One notable example is the use of machine learning algorithms by intelligence agencies to analyze social media patterns. By monitoring platforms like Twitter and Facebook, analysts can identify potential threats based on specific keywords, hashtags, and user behaviors. This proactive approach allows agencies to pinpoint individuals who may be planning harmful activities, often before they even realize they are on the radar.
Another compelling case occurred in the United States, where a combination of predictive analytics and real-time data processing was employed to thwart a planned attack. By analyzing historical data from previous incidents, authorities developed a predictive model that assessed the likelihood of certain locations being targeted. When a spike in suspicious activity was detected in a particular area, law enforcement was able to mobilize resources and prevent an attack from happening. This intervention not only highlighted the effectiveness of data analytics but also underscored the importance of timely action based on the insights gleaned from data.
Furthermore, international collaborations have also benefited from data analytics. For instance, the European Union has implemented a shared data analytics platform that allows member states to exchange information regarding potential threats. This platform utilizes big data frameworks to analyze diverse datasets, including travel records and financial transactions. By doing so, authorities can identify patterns that suggest terrorist financing or movement, leading to early interventions that disrupt plots before they can be executed.
To illustrate these successful interventions, consider the following table that summarizes key cases where data analytics played a crucial role:
Case Study | Methodology Used | Outcome |
---|---|---|
Social Media Monitoring | Machine Learning Algorithms | Identification of potential threats before attacks were planned |
Predictive Analytics in the U.S. | Historical Data Analysis | Thwarted a planned attack through timely intervention |
EU Collaborative Platform | Big Data Frameworks | Disruption of terrorist financing and movements |
These examples showcase the transformative impact of data analytics in counter-terrorism operations. By leveraging technology to analyze vast amounts of data, agencies can act swiftly and effectively to neutralize threats. The key takeaway here is that the integration of data analytics into counter-terrorism strategies not only enhances situational awareness but also fosters a proactive stance against potential attacks. As we continue to advance our analytical capabilities, the potential for successful interventions will only grow, providing a safer environment for everyone.
- What is the primary benefit of using data analytics in counter-terrorism? Data analytics enables agencies to identify patterns and predict potential threats, allowing for timely interventions that can prevent attacks.
- How do machine learning algorithms contribute to counter-terrorism efforts? Machine learning algorithms analyze vast datasets to identify suspicious behaviors and trends, helping authorities to pinpoint potential threats before they escalate.
- What ethical concerns arise from the use of data analytics in national security? The use of data analytics raises questions about privacy and civil liberties, necessitating a careful balance between security measures and individual rights.
- Can data analytics be used in real time? Yes, real-time data processing allows agencies to analyze information as it comes in, which is crucial for thwarting imminent threats.
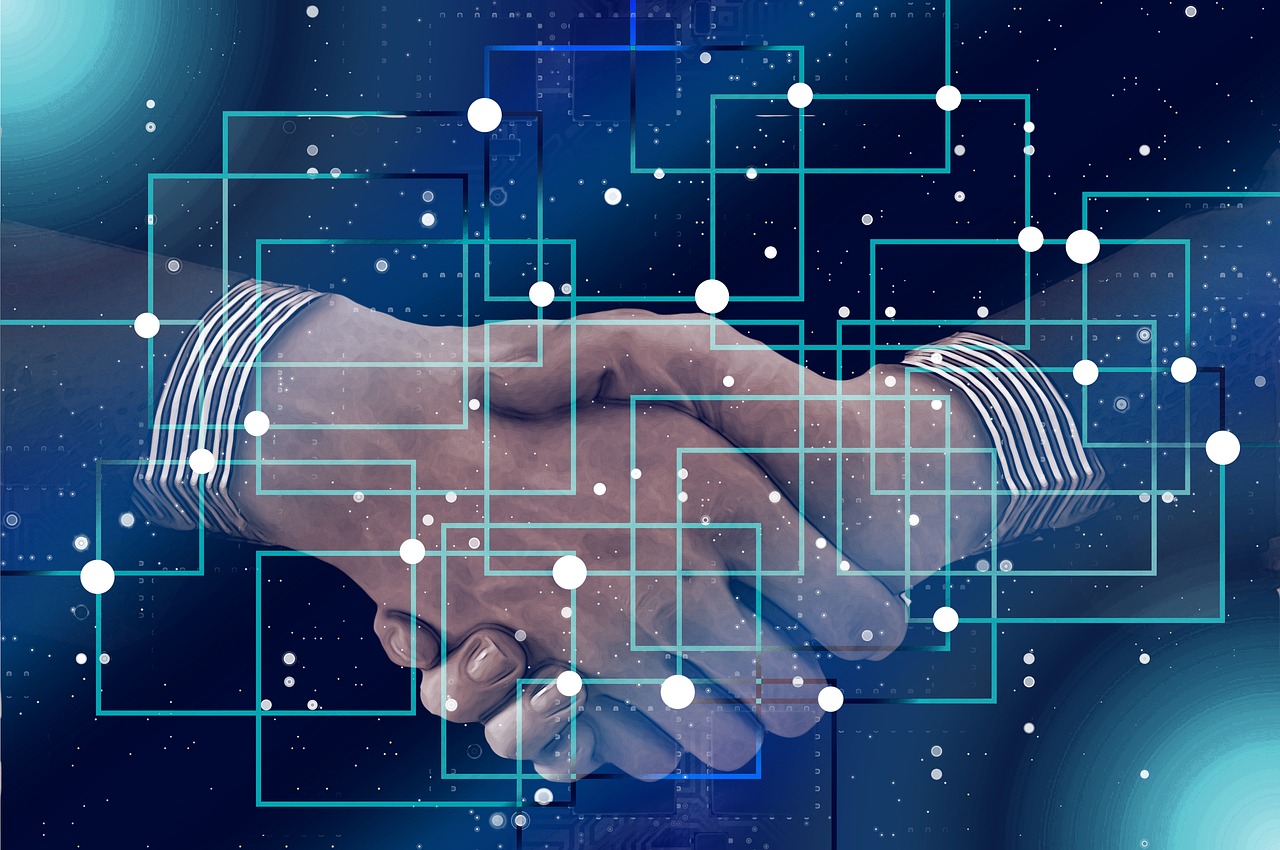
Lessons Learned
In the ever-evolving landscape of counter-terrorism, data analytics has emerged as a pivotal tool that not only enhances operational efficiency but also provides critical insights that can save lives. One of the most significant lessons learned from various operations is the importance of integrating diverse data sources. By analyzing information from multiple channels—such as social media, financial transactions, and public records—agencies can develop a more comprehensive picture of potential threats. For instance, a case study involving the tracking of financial patterns revealed that unusual spending habits often preceded terrorist activities, allowing authorities to intervene before an attack could occur.
Another crucial takeaway is the necessity of collaboration among agencies. The sharing of intelligence and data across different organizations can lead to more effective outcomes. When agencies work in silos, critical information can be missed, which may result in devastating consequences. Effective collaboration not only enhances the quality of data analysis but also fosters a culture of trust among stakeholders, which is essential for national security.
Moreover, the importance of real-time data processing cannot be overstated. The ability to analyze data as it comes in enables agencies to act swiftly, potentially thwarting terrorist plots before they materialize. For example, during a recent operation, the real-time monitoring of social media platforms allowed analysts to detect and respond to a planned attack within hours. This immediacy is what makes data analytics a game-changer in counter-terrorism efforts.
However, these lessons are not without challenges. The need for skilled analysts is paramount. Even the most sophisticated data analytics tools are ineffective without human expertise to interpret the data accurately. Training programs that focus on developing analytical skills among personnel are essential to ensure that agencies can fully leverage the capabilities of data analytics.
Lastly, as we continue to harness the power of data analytics, it is vital to maintain a balance between security and individual rights. The ethical considerations surrounding data privacy must be at the forefront of any counter-terrorism strategy. Agencies must ensure that their methods do not infringe upon civil liberties, which can lead to public distrust and resistance against necessary security measures.
- What is data analytics in counter-terrorism?
Data analytics in counter-terrorism refers to the process of examining and interpreting vast amounts of data to identify patterns, trends, and potential threats related to terrorist activities. - How does machine learning contribute to counter-terrorism?
Machine learning algorithms analyze historical data to predict and identify potential threats, allowing agencies to focus their resources on high-risk areas. - What are the ethical concerns related to data analytics?
Ethical concerns include privacy issues and the potential for misuse of data, which can infringe on individual rights and civil liberties. - Why is real-time data processing important?
Real-time data processing allows agencies to respond immediately to emerging threats, significantly increasing the chances of preventing terrorist activities.
Frequently Asked Questions
- What is data analytics in the context of counter-terrorism?
Data analytics refers to the process of examining raw data to identify patterns and gain insights, which is crucial in national security. In counter-terrorism, it helps agencies analyze vast amounts of information to detect potential threats before they escalate.
- How does machine learning contribute to counter-terrorism efforts?
Machine learning algorithms can sift through enormous datasets to identify suspicious activities or individuals. By learning from historical data, these algorithms improve their accuracy over time, allowing agencies to predict and prevent potential terrorist actions more effectively.
- What are some key technologies used in data analytics?
Technologies such as artificial intelligence, big data frameworks, and predictive analytics are pivotal in enhancing data analytics. They enable real-time processing of information and provide agencies with tools to forecast threats based on past behaviors.
- What types of data are analyzed for counter-terrorism?
Counter-terrorism efforts utilize a variety of data sources, including social media activity, surveillance footage, public records, and communication logs. Analyzing this diverse data helps in building a comprehensive picture of potential threats.
- What challenges does data analytics face in counter-terrorism?
Some significant challenges include data privacy concerns, the risk of information overload, and the necessity for skilled analysts who can interpret complex datasets accurately. Balancing security needs with individual rights is also a major hurdle.
- Are there ethical considerations in using data analytics for security?
Yes, the use of data analytics in counter-terrorism raises ethical questions regarding privacy and civil liberties. It’s essential to find a balance between implementing effective security measures and respecting individuals' rights.
- How important is data quality in counter-terrorism analytics?
Data quality is critical for accurate analysis. Poor-quality data can lead to incorrect conclusions and potentially disastrous decisions in counter-terrorism operations, making it essential to ensure data integrity.
- Can you provide examples of successful data analytics interventions?
Yes, there have been several notable cases where data analytics has led to successful interventions, such as thwarting planned attacks through predictive modeling and real-time data analysis. These examples highlight the effectiveness of data-driven strategies in enhancing national security.
- What lessons have been learned from past counter-terrorism operations?
Analyzing previous operations has provided valuable insights, such as the importance of data collaboration among agencies, the need for continuous training in data analytics, and the effectiveness of proactive threat detection strategies.